Extremely dark light object detection method based on RAW image
An object detection and image technology, applied in the field of computational vision, can solve problems such as difficult labeling, time-consuming, complex noise, etc., achieve the effect of saving image collection resources and human resources, ensuring stability and robustness, and expanding application scenarios
- Summary
- Abstract
- Description
- Claims
- Application Information
AI Technical Summary
Problems solved by technology
Method used
Image
Examples
Embodiment
[0068] In order to illustrate the effects of the present invention, this embodiment will compare various methods under the same experimental conditions.
[0069] 1. Experimental conditions
[0070] The hardware test conditions of this experiment are: the GPU is P40, and the video memory is 24G. The extremely dim light object detection data used in the test is a real extremely dim light object detection dataset manually marked by experts in the relevant field.
[0071] 2. Experimental results
[0072] The effectiveness of the detection method disclosed in the present invention is verified from multiple angles and in all directions by comparing different extremely dim light object detection schemes.
[0073] Table 1 Comparison of extremely dim light object detection schemes
[0074]
[0075] It can be seen from the results in Table 1 that the method disclosed in the present invention can achieve a very good detection effect based on the existing object detection network. T...
PUM
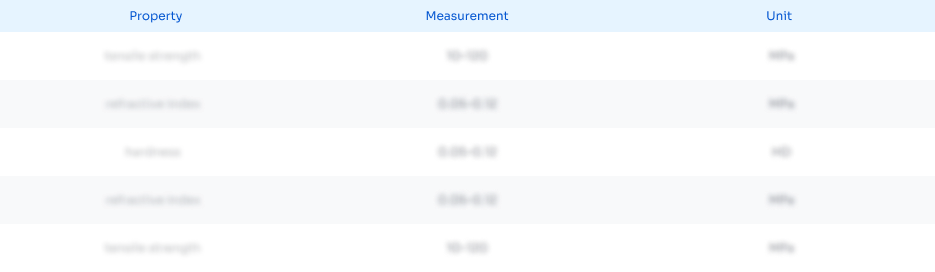
Abstract
Description
Claims
Application Information
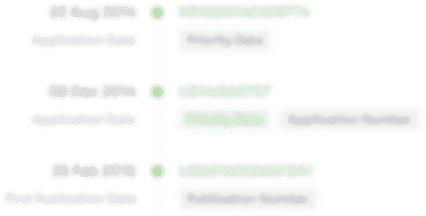
- R&D Engineer
- R&D Manager
- IP Professional
- Industry Leading Data Capabilities
- Powerful AI technology
- Patent DNA Extraction
Browse by: Latest US Patents, China's latest patents, Technical Efficacy Thesaurus, Application Domain, Technology Topic, Popular Technical Reports.
© 2024 PatSnap. All rights reserved.Legal|Privacy policy|Modern Slavery Act Transparency Statement|Sitemap|About US| Contact US: help@patsnap.com