Multi-modal event representation learning method based on event ontology
A technology of event representation and learning method, applied in the field of multi-modal data representation learning, can solve problems such as long semantic distance, lack of semantic information for events, and insufficient expressive ability of single-modal events, so as to enhance semantics and solve limitations Effect
- Summary
- Abstract
- Description
- Claims
- Application Information
AI Technical Summary
Problems solved by technology
Method used
Image
Examples
Embodiment 1
[0104] like figure 1 As shown, the multimodal event representation learning based on event ontology in the present invention may include the following steps:
[0105] Step S101: the construction of the relevant field event ontology and the formation of an event ontology model;
[0106] Step S102: Preprocessing of the initial multimodal event information data, according to the language expression defined in the event ontology, different events are grouped into different event classes.
[0107] Step S103: adopt multimodal pre-training models such as ontology model and VilBERT to embed the multimodal event data;
[0108] Step S104: using graph convolutional neural network models such as GCN and GAT to learn the graph structure composed of events and events and events and relationships in the ontology.
[0109] In step S101, first collect relevant domain texts and build a corpus; by learning the corpus, extract the expressed events and event elements from the domain text content...
Embodiment 2
[0118] On the basis provided by Embodiment 1, the preprocessing of the initial multimodal event information data in step S102, according to the language expression defined in the event ontology, will group different events into different event categories, specifically including the following steps :
[0119] 1. Use Faster-RCNN and other target detection algorithms to extract the object area in the picture information and the feature representation of the area as the image input part of VilBERT;
[0120] 2. Extract the core keywords of an event in the text information and perform cosine similarity calculation and classification according to the language performance defined by the ontology, specifically satisfying the following formula:
[0121]
[0122] Among them, e c Represents the n-dimensional embedded representation of a certain event class, α represents the embedded representation obtained by averaging the event text keywords, Sim(e c ,α) indicates the similarity bet...
Embodiment 3
[0125] On the basis provided in Embodiment 2, the embedding representation of multimodal event data using multimodal pre-training models such as ontology model and VilBERT in step S103 may include the following steps:
[0126] 1. Obtain the trained VilBERT model, and use the regional image feature representation and text information extracted in Example 2 as the input of the model.
[0127] 2. The embedded representation generated by the VilBERT model according to the input data and the embedded representation corresponding to the event class to which it belongs are spliced and fused to obtain a new embedded representation.
PUM
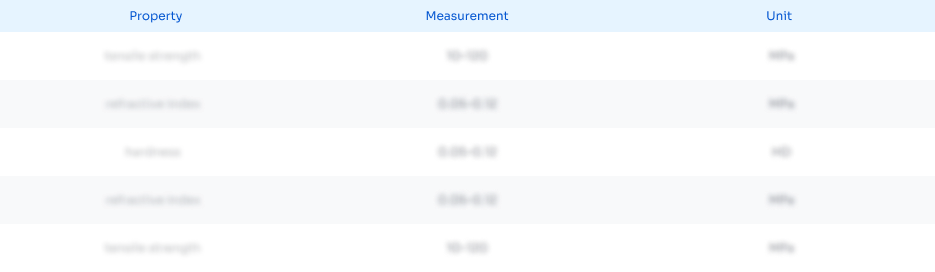
Abstract
Description
Claims
Application Information
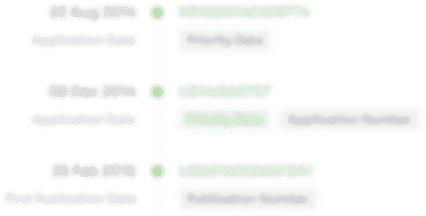
- R&D Engineer
- R&D Manager
- IP Professional
- Industry Leading Data Capabilities
- Powerful AI technology
- Patent DNA Extraction
Browse by: Latest US Patents, China's latest patents, Technical Efficacy Thesaurus, Application Domain, Technology Topic, Popular Technical Reports.
© 2024 PatSnap. All rights reserved.Legal|Privacy policy|Modern Slavery Act Transparency Statement|Sitemap|About US| Contact US: help@patsnap.com