Software-defined space-air-ground integrated network routing optimization method based on deep reinforcement learning
A reinforcement learning and software-defined technology, applied in the field of wireless communication, can solve problems such as poor link quality, high hardware requirements for dynamic topology routing algorithms, and large node resource occupation, and achieve average end-to-end delay and throughput Improvement, high theoretical value and practical significance, effect of improving stability and reliability
- Summary
- Abstract
- Description
- Claims
- Application Information
AI Technical Summary
Problems solved by technology
Method used
Image
Examples
Embodiment Construction
[0013] Attached below figure 1 The process flow is described in detail for a specific implementation of a software-defined air-space-ground integrated network routing optimization method based on deep reinforcement learning in the present invention.
[0014] like figure 1 As shown, the present invention provides a software-defined air-space-ground integrated network routing optimization method based on deep reinforcement learning, including:
[0015] Step 1: Build a software-defined air-space-ground integrated network topology according to the software-defined network idea and the parameters of the air-space-ground integrated network nodes, and initialize the topology discovery module, network perception module and routing decision-making module.
[0016] Step 2: Through the topology discovery module and network perception module initialized in step 1, the controller monitors the network topology in the current state and the state data of each link in the current network, and...
PUM
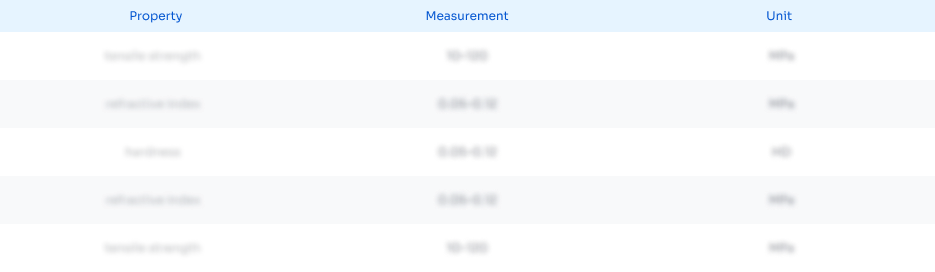
Abstract
Description
Claims
Application Information
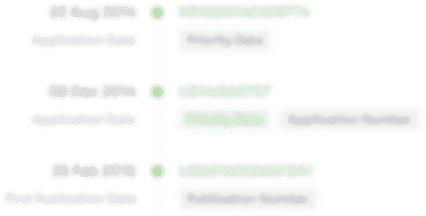
- R&D Engineer
- R&D Manager
- IP Professional
- Industry Leading Data Capabilities
- Powerful AI technology
- Patent DNA Extraction
Browse by: Latest US Patents, China's latest patents, Technical Efficacy Thesaurus, Application Domain, Technology Topic, Popular Technical Reports.
© 2024 PatSnap. All rights reserved.Legal|Privacy policy|Modern Slavery Act Transparency Statement|Sitemap|About US| Contact US: help@patsnap.com