Rolling bearing fault diagnosis method based on full convolution auto-encoder and optimized support vector machine
A convolutional self-encoding and support vector machine technology, applied in the field of rolling bearing fault diagnosis, can solve problems such as low efficiency, low fault diagnosis accuracy, and incomplete extraction of effective signal features
- Summary
- Abstract
- Description
- Claims
- Application Information
AI Technical Summary
Problems solved by technology
Method used
Image
Examples
Embodiment Construction
[0020] (1) Rolling bearing data preprocessing
[0021] The invention adopts the rolling bearing vibration data collected by the Bearing Experiment Center of Case Western Reserve University in the United States to carry out experiments. The data of 10 types of faults with different fault degrees including normal, inner ring fault, rolling element fault and outer ring fault were selected. Each type of data is divided into multiple samples of equal length, and the length of a single sample is set to 500, which ensures that each sample contains all the vibration information of one revolution of the bearing. The bearing vibration signal of each fault type is divided into 240 samples, 200 samples are randomly selected as training data, and the remaining 40 samples are used as test data, that is, the training data set contains a total of 2000 samples, and the test data set contains a total of 400 samples. Fourier transform is used to convert all samples from time-domain signal to fr...
PUM
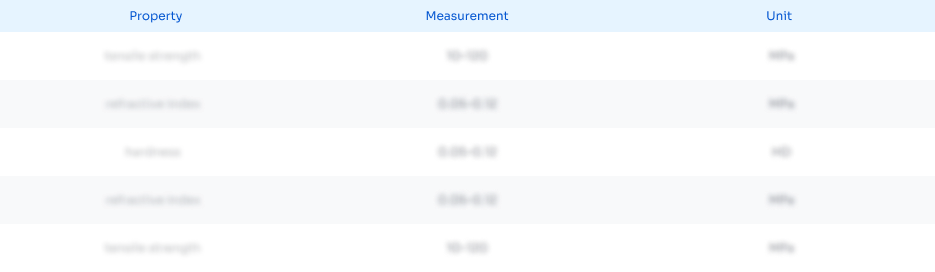
Abstract
Description
Claims
Application Information
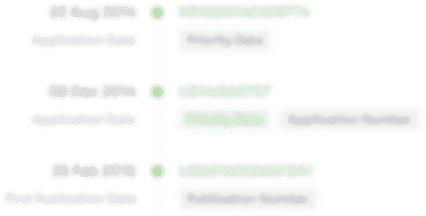
- R&D Engineer
- R&D Manager
- IP Professional
- Industry Leading Data Capabilities
- Powerful AI technology
- Patent DNA Extraction
Browse by: Latest US Patents, China's latest patents, Technical Efficacy Thesaurus, Application Domain, Technology Topic.
© 2024 PatSnap. All rights reserved.Legal|Privacy policy|Modern Slavery Act Transparency Statement|Sitemap