Machine learning multispectral remote sensing image crop straw out-of-field extraction method and system
A multi-spectral image and machine learning technology, applied in the field of multi-spectral satellite remote sensing image data mining, can solve the problems of measurement error, human error of area and progress, and regional positioning control that cannot leave the field, so as to reduce costs and solve efficiency. Low, the effect of preventing illegal burning
- Summary
- Abstract
- Description
- Claims
- Application Information
AI Technical Summary
Problems solved by technology
Method used
Image
Examples
Embodiment approach 1
[0044] Embodiment 1. The method for extracting crop stalks from field plots of multi-spectral remote sensing images based on machine learning described in this embodiment, refer to figure 1 This embodiment can be better understood, including the following steps:
[0045] Step S1, obtain the multi-spectral image data of the crop growth period, outline and establish a polygonal sample set according to the crop spectral information, establish a crop classification model, and predict the crop distribution result after the establishment of the crop classification model;
[0046] Step S2, obtaining the multispectral image data after the harvest period of the crops, drawing polygonal samples of the corresponding category, and performing sampling processing to obtain a polygonal sample set of off-field plots;
[0047] Step S3, using the 50% division to divide the polygonal sample set of the field plot, and the obtained five sets of training sets-verification sets are trained as five b...
Embodiment approach 2
[0050]Embodiment 2. This embodiment is a method for extracting crop stalks and field plots from multi-spectral remote sensing images of machine learning described in Embodiment 1. In this embodiment, the multispectral data obtained in the step S1 of the crop growth period In the image data, the multi-spectral image data refers to the wave band as:
[0051] The reflectance data of 10 bands including B2, B3, B4, B5, B6, B7, B8, B8A, B11, and B12, and the multispectral image data format is 16-bit unsigned integer.
[0052] In this embodiment, the reflectance products of 10 bands are selected for the multi-spectral image. The reason is that there are certain differences in the spectral characteristics of the off-field and non-off-field in this band, and the machine learning algorithm used in this method is more sensitive to multi-features. A good effect can automatically reduce the weight of features that do not improve the accuracy of the model. By extracting the features of the...
Embodiment approach 3
[0053] Embodiment 3. This embodiment is a method for extracting crop stalks and field plots from multi-spectral remote sensing images of machine learning described in Embodiment 1. In this embodiment, the steps described in step S1 are to draw and establish polygons based on crop spectral information. sample set,
[0054] The extracted crops include: corn, rice, other crops, and other four types of crops,
[0055] Delineating and establishing a polygonal sample set refers to delineating and establishing 50 to 100 polygonal samples to form a sample set for each category of crops mentioned above.
[0056] In this embodiment, the band feature value of each pixel is extracted according to the sketched sample polygon as a training set.
PUM
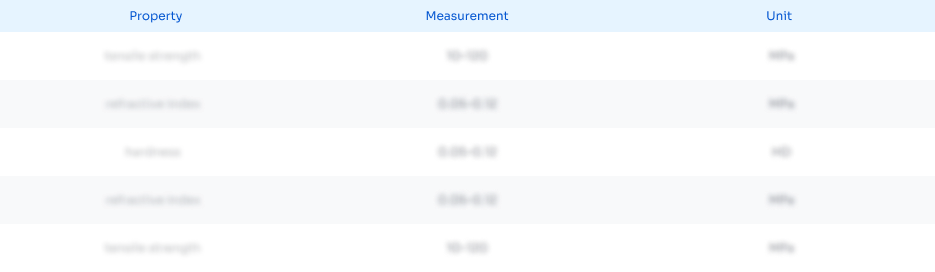
Abstract
Description
Claims
Application Information
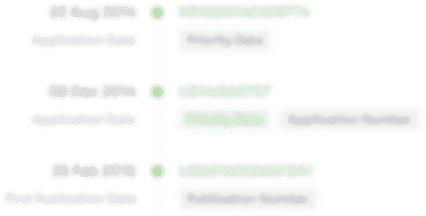
- R&D Engineer
- R&D Manager
- IP Professional
- Industry Leading Data Capabilities
- Powerful AI technology
- Patent DNA Extraction
Browse by: Latest US Patents, China's latest patents, Technical Efficacy Thesaurus, Application Domain, Technology Topic.
© 2024 PatSnap. All rights reserved.Legal|Privacy policy|Modern Slavery Act Transparency Statement|Sitemap