Lithium battery residual life prediction method
A life prediction, lithium battery technology, applied in the measurement of electricity, measurement devices, electric vehicles and other directions, can solve the problems affecting the accuracy of the model, affecting the accuracy of lithium batteries, health factor redundancy, etc., to reduce the amount of training, reduce selection time , to solve the effect of redundancy
- Summary
- Abstract
- Description
- Claims
- Application Information
AI Technical Summary
Problems solved by technology
Method used
Image
Examples
Embodiment Construction
[0035] The present invention is a lithium battery remaining life prediction method based on random forest health factor selection and Bayesian optimization BiLSTM:
[0036] Step 1: Offline modeling, collecting offline data such as current, voltage, temperature, etc. during the charging and discharging process of lithium batteries, and obtaining a sample set of health factors by extracting lithium battery degradation feature sequences. Then use the random forest algorithm to analyze the weight of the health factor sample set, and determine the selected health factor sample according to the weight. The selected samples are added to the training set in order, and the initialization parameters are randomly generated and substituted into the BiLSTM model for training. When the accuracy of the training results does not meet the requirements, a Gaussian process regression model is established to obtain the expression of the collection function, and the next set of model parameters is ...
PUM
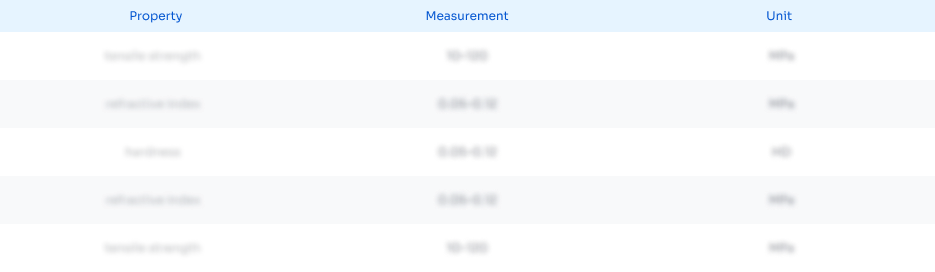
Abstract
Description
Claims
Application Information
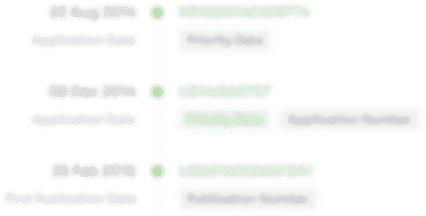
- R&D Engineer
- R&D Manager
- IP Professional
- Industry Leading Data Capabilities
- Powerful AI technology
- Patent DNA Extraction
Browse by: Latest US Patents, China's latest patents, Technical Efficacy Thesaurus, Application Domain, Technology Topic, Popular Technical Reports.
© 2024 PatSnap. All rights reserved.Legal|Privacy policy|Modern Slavery Act Transparency Statement|Sitemap|About US| Contact US: help@patsnap.com