Deep counterfeit image detection method fusing depth learning and width learning
An image detection and grayscale image technology, which is applied in the field of deep fake image detection integrating depth and width learning, can solve the problems of inability to handle fake images, poor feature representation ability and method generalization, and achieves overcoming the random generation of a large number of weight matrices. , save computing costs, avoid the effect of unstable results
- Summary
- Abstract
- Description
- Claims
- Application Information
AI Technical Summary
Problems solved by technology
Method used
Image
Examples
Embodiment 1
[0074] This embodiment takes a deep fake face image as an example to describe a deep fake image detection method that combines depth and width learning, including the following steps:
[0075] The first step, data preparation; this embodiment is based on the real face images of the CelebA-HQ and CelebA datasets, and generates deep fake face images through the methods of StarGAN, StyleGAN, StyleGAN2, AttGAN, and FaceForensics++ respectively, and five training sets are obtained. and five test sets, each training set and test set respectively contain 1500 and 500 deep fake face images; FaceForensics++ is open source fake face video data, this embodiment uses the single frame face image of its fake video as deep fake face images, to show that the method of the present invention is also applicable to deep forgery face videos; all images are converted into grayscale images with a size of 256*256 pixels.
[0076] The second step is to use the frequency domain feature extraction modul...
PUM
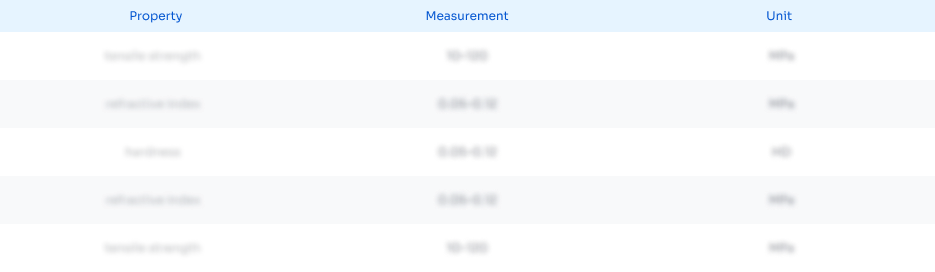
Abstract
Description
Claims
Application Information
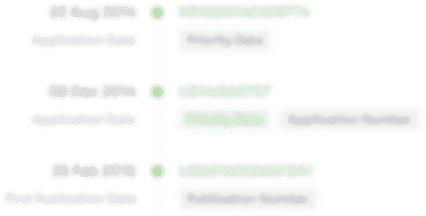
- R&D Engineer
- R&D Manager
- IP Professional
- Industry Leading Data Capabilities
- Powerful AI technology
- Patent DNA Extraction
Browse by: Latest US Patents, China's latest patents, Technical Efficacy Thesaurus, Application Domain, Technology Topic, Popular Technical Reports.
© 2024 PatSnap. All rights reserved.Legal|Privacy policy|Modern Slavery Act Transparency Statement|Sitemap|About US| Contact US: help@patsnap.com