Multi-agent reinforcement learning method and system based on value decomposition
A multi-agent, reinforcement learning technology, applied in neural learning methods, biological models, indoor games, etc., can solve the problems of positive feedback without rewards, difficult to guarantee convergence time, underestimation of rewards, etc., and achieve rewards The effect of stable value, accelerated exploration efficiency, and improved algorithm performance
- Summary
- Abstract
- Description
- Claims
- Application Information
AI Technical Summary
Problems solved by technology
Method used
Image
Examples
Embodiment Construction
[0042] The present invention will be further described in detail below with reference to the accompanying drawings and specific implementations. It should be noted that the following embodiments are intended to facilitate the understanding of the idea of the present invention, but do not have any limiting effect on it.
[0043] The present invention proposes a multi-agent reinforcement learning method based on value decomposition, which can be applied in complex partially observable scenes, such as video games, sensor networks, robot swarm coordination, and autonomous vehicles. Each agent plays a different role in different multi-agent scenarios, such as acting as a hero in a game scenario, representing each sensor in a sensor network, representing a single robot in a robot collaboration scenario, acting as a car in an autonomous driving scenario, Any object that can perceive the environment and can independently make decisions to affect the environment can be abstracted as a...
PUM
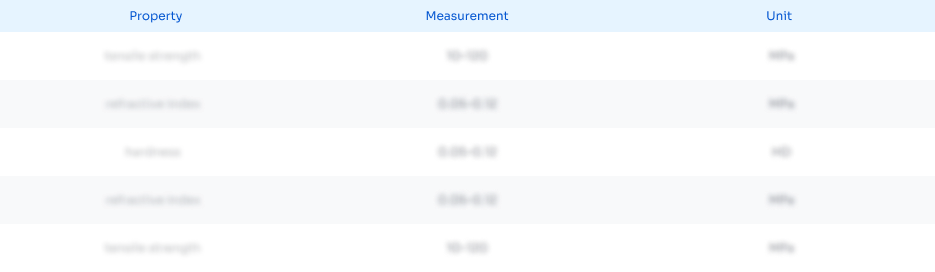
Abstract
Description
Claims
Application Information
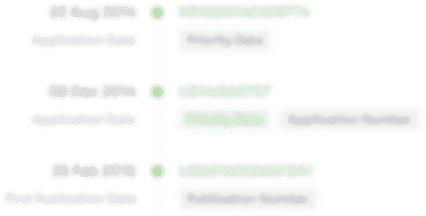
- R&D
- Intellectual Property
- Life Sciences
- Materials
- Tech Scout
- Unparalleled Data Quality
- Higher Quality Content
- 60% Fewer Hallucinations
Browse by: Latest US Patents, China's latest patents, Technical Efficacy Thesaurus, Application Domain, Technology Topic, Popular Technical Reports.
© 2025 PatSnap. All rights reserved.Legal|Privacy policy|Modern Slavery Act Transparency Statement|Sitemap|About US| Contact US: help@patsnap.com