Zero-sample unsupervised image defogging method and system
An unsupervised, image-based technology, applied in the field of image processing, can solve the problems of unsupervised, shortening the inference time of a single image, etc., to reduce the inference time, improve the dehazing performance, and solve the effect of excessive inference time
- Summary
- Abstract
- Description
- Claims
- Application Information
AI Technical Summary
Problems solved by technology
Method used
Image
Examples
Embodiment 1
[0069] like figure 1 As shown, this embodiment provides a zero-sample unsupervised image dehazing method, including the following steps:
[0070] Get a foggy image and use it directly as an input image;
[0071] Construct a zero-sample unsupervised dehazing neural network. The zero-sample unsupervised dehazing neural network includes the atmospheric illumination image prediction branch, the transmission image prediction branch and the haze-free image prediction branch, which are used to realize the layer de-separation of the input fog image and obtain the atmosphere. Light intensity image information, transmission image information, and fog-free image information, which can be continuously updated to obtain the best dehazing image by reconstructing the fog image and calculating constraints;
[0072] The zero-sample unsupervised dehazing neural network is trained as follows: the input required for model training is the learning rate, loss function parameters, the number of ite...
Embodiment 2
[0120] like Figure 5 As shown, this embodiment provides a zero-sample unsupervised image dehazing system, including: a hazy image acquisition module, a zero-sample unsupervised dehazing neural network building module, a zero-sample unsupervised dehazing neural network training module, a loop Iteration module and output module;
[0121] In this embodiment, the foggy image acquisition module is used to acquire a foggy image as an input image;
[0122] In this embodiment, the zero-sample unsupervised dehazing neural network building module is used to construct a zero-sample unsupervised dehazing neural network, and the zero-sample unsupervised dehazing neural network includes an atmospheric illumination image prediction branch, a transmission image prediction branch and a haze-free neural network. image prediction branch;
[0123] In this embodiment, the atmospheric illumination image prediction branch adopts a variational autoencoder structure;
[0124] In this embodiment, t...
PUM
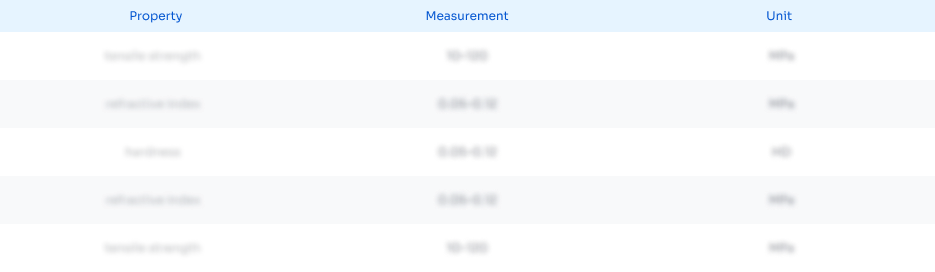
Abstract
Description
Claims
Application Information
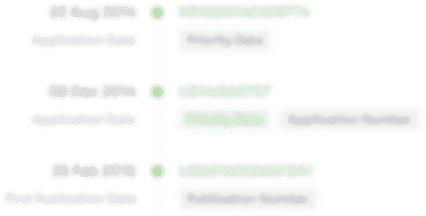
- R&D Engineer
- R&D Manager
- IP Professional
- Industry Leading Data Capabilities
- Powerful AI technology
- Patent DNA Extraction
Browse by: Latest US Patents, China's latest patents, Technical Efficacy Thesaurus, Application Domain, Technology Topic.
© 2024 PatSnap. All rights reserved.Legal|Privacy policy|Modern Slavery Act Transparency Statement|Sitemap