Eye fundus photo classification method and eye fundus image processing method and system
A classification method and photo technology, applied in the field of medical image classification and detection, can solve the problems of low accuracy and low efficiency, and achieve the effect of high effect, solving overfitting and occupying less computer resources.
- Summary
- Abstract
- Description
- Claims
- Application Information
AI Technical Summary
Problems solved by technology
Method used
Image
Examples
Embodiment 1
[0089] The fundus photo classification method described in the embodiment of the present invention mainly uses image morphology and deep learning methods, uses the morphological method to preprocess the fundus photos, and then introduces a more suitable The focal loss loss function for the incidence problem and the training strategy for the class imbalance problem, an automatic classification method based on the DenseNet-101 network model is designed.
[0090] To achieve the above purpose of the invention, an embodiment of the present invention proposes a method for image preprocessing and automatic classification of fundus photos based on a deep learning algorithm, including the following steps:
[0091] (1) Through the preprocessing of the fundus photo, a standardized fundus photo is generated, such as figure 2 shown
[0092] 1.15) For each fundus photo, first extract the region of interest (ROI), extract the R channel component of the fundus photo, and binarize it;
[00...
Embodiment 2
[0143] In the prior art, a convolutional neural network model using an attention branch module is used to process fundus image data, but the attention branch module still has many deficiencies. First of all, the attention branch module refers to the practice of CAM, and replaces the fully connected layer connected with softmax to GAP. Compared with the fully connected layer that can be trained with parameters, GAP is only a pooling operation and cannot be adjusted, and the previous layer is added. The training pressure of the convolutional layer leads to slower global convergence. Secondly, the attention branch module receives the feature map output from the previous convolution layer, and compresses the feature map information into CAM through ordinary convolution and 1x1x1 convolution; on the other branch, the attention branch module uses 1x1 convolution and The GAP method constrains the loss function. In fact, the two components of 1x1 convolution and GAP can directly expor...
Embodiment approach
[0145] Specifically, see Figure 5 , according to a preferred embodiment of the present invention, a fundus image processing method is provided, which is characterized by comprising the following steps:
[0146] Constructing the first data set, including the preprocessing of the original fundus image and the data screening of the preprocessed fundus image, the preprocessing of the original fundus image includes data desensitization, ROI extraction and contrast enhancement, and the preprocessed fundus image. The image data screening includes using the MobileNet v3 large model and the soft voting method to screen the preprocessed fundus images, clearing the fundus images with substandard quality, and constructing the first data set;
[0147]Building a convolutional neural network model with a CCAM module, the convolutional neural network model comprising a backbone network and the CCAM module, the backbone network comprising an input, a first convolutional layer, an MLP and a fi...
PUM
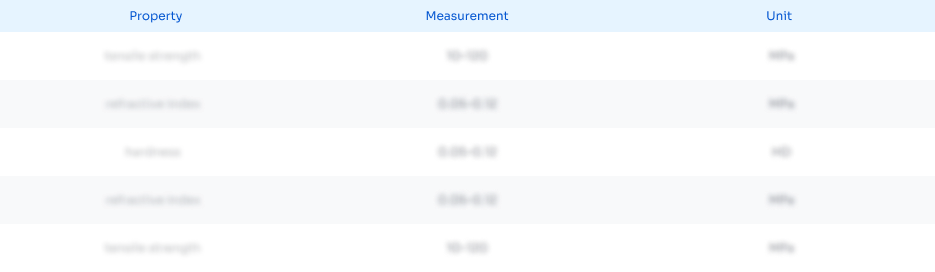
Abstract
Description
Claims
Application Information
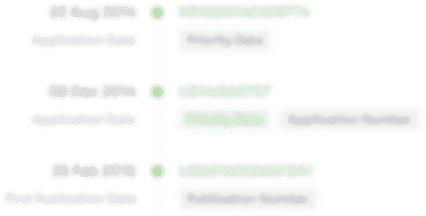
- R&D Engineer
- R&D Manager
- IP Professional
- Industry Leading Data Capabilities
- Powerful AI technology
- Patent DNA Extraction
Browse by: Latest US Patents, China's latest patents, Technical Efficacy Thesaurus, Application Domain, Technology Topic, Popular Technical Reports.
© 2024 PatSnap. All rights reserved.Legal|Privacy policy|Modern Slavery Act Transparency Statement|Sitemap|About US| Contact US: help@patsnap.com