Electric vehicle lithium battery residual life prediction method based on XGBoost-LSTM optimization model
A technology for electric vehicles and life prediction, applied in the direction of measuring electricity, measuring devices, measuring electrical variables, etc., can solve problems such as limitations, increased RUL prediction time cost and complexity, and inaccurate prediction models to achieve high-precision prediction , long-term forecasting performance improvement, and the effect of forecasting accuracy improvement
- Summary
- Abstract
- Description
- Claims
- Application Information
AI Technical Summary
Problems solved by technology
Method used
Image
Examples
Embodiment Construction
[0021] The technical solutions in the embodiments of the present invention will be clearly and completely described below with reference to the accompanying drawings in the embodiments of the present invention. Obviously, the described embodiments are only a part of the embodiments of the present invention, not all of the embodiments. The embodiments of the present invention, and all other embodiments obtained by those of ordinary skill in the art without creative work, fall within the protection scope of the present invention.
[0022] see Figure 1-3 , a method for predicting the remaining life of an electric vehicle lithium battery based on the XGBoost-LSTM optimization model, comprising the following steps:
[0023] (1) First, based on the online collection technology of electric vehicle lithium battery information, collect the charging data of the electric vehicle lithium battery on the can bus of the charging pile. If the collected electric vehicle lithium battery chargi...
PUM
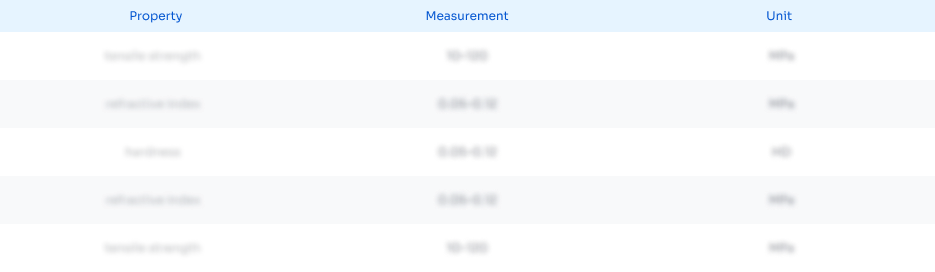
Abstract
Description
Claims
Application Information
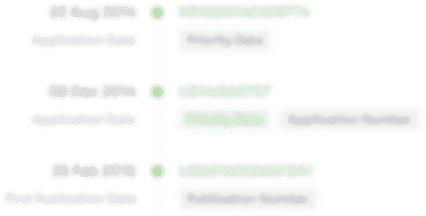
- R&D Engineer
- R&D Manager
- IP Professional
- Industry Leading Data Capabilities
- Powerful AI technology
- Patent DNA Extraction
Browse by: Latest US Patents, China's latest patents, Technical Efficacy Thesaurus, Application Domain, Technology Topic, Popular Technical Reports.
© 2024 PatSnap. All rights reserved.Legal|Privacy policy|Modern Slavery Act Transparency Statement|Sitemap|About US| Contact US: help@patsnap.com