Bearing cross-working-condition fault prediction method based on adversarial transfer learning
A technology of transfer learning and fault prediction, applied in neural learning methods, instruments, biological neural network models, etc., can solve problems such as difficult maintenance, difficult to predict remaining life, economic loss, etc., and achieve strong robustness and generalization ability, excellent migration prediction performance, and the effect of resolving significant distribution differences
- Summary
- Abstract
- Description
- Claims
- Application Information
AI Technical Summary
Problems solved by technology
Method used
Image
Examples
Embodiment Construction
[0038] The present invention will be further described in detail below with reference to the accompanying drawings and specific embodiments. The numbers of the steps in the following embodiments are only set for the convenience of description, and the sequence between the steps is not limited in any way, and the execution sequence of each step in the embodiments can be adapted according to the understanding of those skilled in the art Sexual adjustment.
[0039] refer to figure 1 and figure 2 , the present invention provides a bearing fault prediction method based on adversarial transfer learning across working conditions, the method includes the following steps:
[0040] S1. Set different working conditions and collect signals from the bearing to obtain vibration signals;
[0041] S1.1. Collect the bearing vibration signal of the equipment running to the cut-off point, and obtain the fault characteristic data of the target domain;
[0042] S1.2. Run the same equipment fr...
PUM
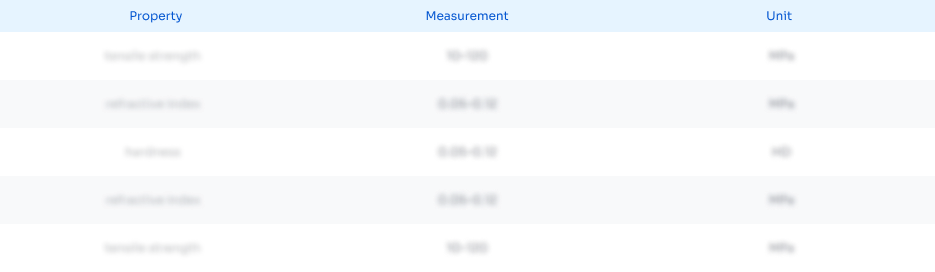
Abstract
Description
Claims
Application Information
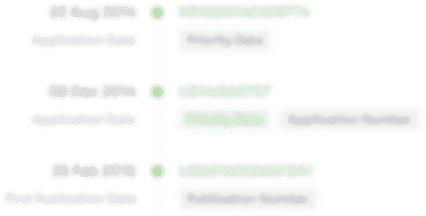
- R&D Engineer
- R&D Manager
- IP Professional
- Industry Leading Data Capabilities
- Powerful AI technology
- Patent DNA Extraction
Browse by: Latest US Patents, China's latest patents, Technical Efficacy Thesaurus, Application Domain, Technology Topic, Popular Technical Reports.
© 2024 PatSnap. All rights reserved.Legal|Privacy policy|Modern Slavery Act Transparency Statement|Sitemap|About US| Contact US: help@patsnap.com