Industrial process small sample fault diagnosis method based on deep optimal feature transmission
An optimal feature and fault diagnosis technology, applied in the direction of comprehensive factory control, instrumentation, adaptive control, etc., can solve the problems of lack of incremental learning mechanism in domain adaptation methods, achieve short calculation cycle, low algorithm complexity, and High transplantability effect
- Summary
- Abstract
- Description
- Claims
- Application Information
AI Technical Summary
Problems solved by technology
Method used
Image
Examples
Embodiment Construction
[0020] The present invention is further described below with reference to the accompanying drawings and specific embodiments, but does not limit the scope of the present invention in any way.
[0021] like figure 1 As shown, the small-sample fault diagnosis method based on deep optimal feature transmission provided by the present invention is mainly divided into four steps: constructing a fault adaptation task set, characterizing the task set in the embedding space, and modeling the paradigm learned in the embedding space. Identification and parameter estimation, updating the model transmission depth optimal features, and obtaining the fault classification results under unknown tasks.
[0022] The invention constructs a small-sample fault diagnosis network model: including an adaptive network model and a non-adaptive network model; the adaptive network model can include multi-layer networks, which are respectively used for fixing through the training of the source domain, and ...
PUM
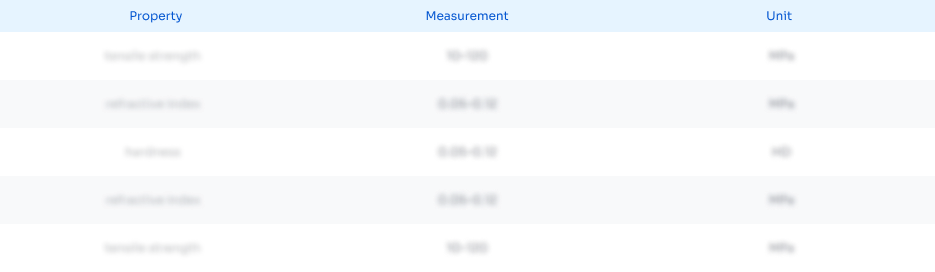
Abstract
Description
Claims
Application Information
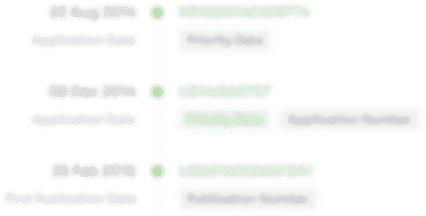
- R&D
- Intellectual Property
- Life Sciences
- Materials
- Tech Scout
- Unparalleled Data Quality
- Higher Quality Content
- 60% Fewer Hallucinations
Browse by: Latest US Patents, China's latest patents, Technical Efficacy Thesaurus, Application Domain, Technology Topic, Popular Technical Reports.
© 2025 PatSnap. All rights reserved.Legal|Privacy policy|Modern Slavery Act Transparency Statement|Sitemap|About US| Contact US: help@patsnap.com