Magnetic resonance reconstruction method of super-resolution convolutional neural network based on cavity convolution
A convolutional neural network and super-resolution technology, applied in the field of magnetic resonance reconstruction, can solve the problems of uncomfortable patients and long duration of MR imaging, and achieve the effects of improved detail recovery, improved effectiveness, and improved performance
- Summary
- Abstract
- Description
- Claims
- Application Information
AI Technical Summary
Problems solved by technology
Method used
Image
Examples
Embodiment Construction
[0027] The present invention will be described in detail below with reference to the accompanying drawings and specific embodiments.
[0028] An embodiment provided by the present invention: a magnetic resonance reconstruction method based on a hole convolution super-resolution convolutional neural network, inspired by the classic super-resolution algorithm SRCNN, and then designed an end-to-end convolutional neural network network to complete magnetic resonance image reconstruction. Specifically include the following steps:
[0029] In step 1, we select 150 MRI images from the dataset as the training set, and the image size is 256*256.
[0030] Step 2: Introduce SRCNN, which is considered to be the first super-resolution reconstruction method using the convolutional neural network structure. In this method, for a low-resolution image, it first uses bicubic interpolation to enlarge it to the target size, and then performs nonlinear mapping through a three-layer convolutional...
PUM
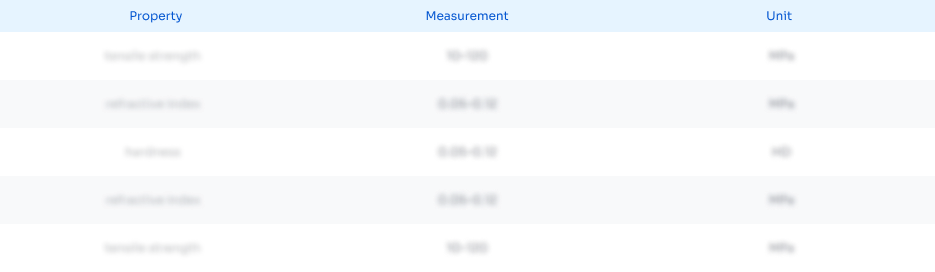
Abstract
Description
Claims
Application Information
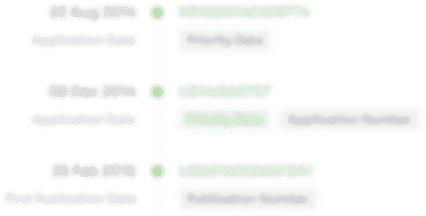
- Generate Ideas
- Intellectual Property
- Life Sciences
- Materials
- Tech Scout
- Unparalleled Data Quality
- Higher Quality Content
- 60% Fewer Hallucinations
Browse by: Latest US Patents, China's latest patents, Technical Efficacy Thesaurus, Application Domain, Technology Topic, Popular Technical Reports.
© 2025 PatSnap. All rights reserved.Legal|Privacy policy|Modern Slavery Act Transparency Statement|Sitemap|About US| Contact US: help@patsnap.com