Deep learning data privacy protection method, system and device and medium
A deep learning and data privacy technology, applied in the field of deep learning data privacy protection, can solve problems such as unacceptable decline in model performance, lack of comprehensibility, and difficulty in adjustment
- Summary
- Abstract
- Description
- Claims
- Application Information
AI Technical Summary
Problems solved by technology
Method used
Image
Examples
Embodiment 1
[0054] As an aspect of the embodiments of the present disclosure, a deep learning data privacy protection method is provided, such as figure 1 shown, including the following steps:
[0055] S10. Load the original training data set and the deep learning model;
[0056] S20, assigning a privacy protection weight to the privacy information in the original training data set, and constructing a privacy importance matrix;
[0057] S30, configure the global noise intensity and generator parameters in the training data to construct a noise generator; train the noise generator according to the loss function constructed by the privacy importance matrix;
[0058] S40. Add noise to all the original training data in the original training data set through a noise generator to generate a noise-added data set;
[0059] S60. Use the noise-added data set to train the deep learning model to form a deep learning model with privacy protection characteristics.
[0060] Based on the above configu...
Embodiment 2
[0088] As another aspect of the embodiments of the present disclosure, a deep learning data privacy protection system 100 is provided, such as image 3 shown, including:
[0089]Resource loading module 1, loading the original training dataset and deep learning model;
[0090] Privacy importance configuration module 2, assigning privacy protection weights to the privacy information in the original training data set, and constructing a privacy importance matrix;
[0091] Noise generator building module 3, configure the global noise intensity and generator parameters in the training data to construct a noise generator; train the noise generator according to the loss function constructed by the privacy importance matrix;
[0092] The data conversion module 4 is to add noise to all the original training data in the original training data set through the noise generator to generate a noise-added data set;
[0093] The model building module 6 uses the noise-added data set to train ...
Embodiment 3
[0126] An electronic device includes a memory, a processor, and a computer program stored on the memory and running on the processor, where the processor implements the deep learning data privacy protection method in Embodiment 1 when the processor executes the computer program.
[0127] Embodiment 3 of the present disclosure is only an example, and should not impose any limitations on the function and scope of use of the embodiment of the present disclosure.
[0128] The electronic device may take the form of a general-purpose computing device, which may be, for example, a server device. Components of an electronic device may include, but are not limited to, at least one processor, at least one memory, and a bus connecting different system components including memory and processor.
[0129] The bus includes a data bus, an address bus and a control bus.
[0130] The memory may include volatile memory, such as random access memory (RAM) and / or cache memory, and may further inc...
PUM
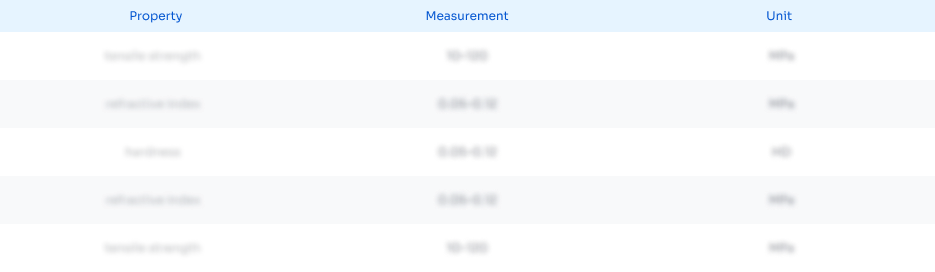
Abstract
Description
Claims
Application Information
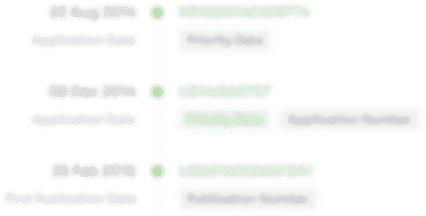
- R&D Engineer
- R&D Manager
- IP Professional
- Industry Leading Data Capabilities
- Powerful AI technology
- Patent DNA Extraction
Browse by: Latest US Patents, China's latest patents, Technical Efficacy Thesaurus, Application Domain, Technology Topic, Popular Technical Reports.
© 2024 PatSnap. All rights reserved.Legal|Privacy policy|Modern Slavery Act Transparency Statement|Sitemap|About US| Contact US: help@patsnap.com