Online variational Gaussian process method for time series data
A Gaussian process, time series technique, used in the computer field
- Summary
- Abstract
- Description
- Claims
- Application Information
AI Technical Summary
Problems solved by technology
Method used
Image
Examples
Embodiment Construction
[0059] The embodiments of the present invention will be further described below with reference to the accompanying drawings.
[0060] The invention provides an online variational Gaussian process method for time series data, including four parts: solving the new relative entropy divergence, solving factor distribution, solving variational free energy and making predictions, such as: figure 1 shown, including the following steps:
[0061] S1. Set the problem model and solution framework.
[0062] A regression model using the Gaussian process framework, where the dataset D consists of an N-dimensional input vector and the corresponding real-valued output The ultimate goal is to predict the posterior probability under the current new observation data. Posterior probability refers to the probability that something has happened, and the reason why it is to be calculated is caused by a certain factor; prior probability refers to the probability that can be obtained before exper...
PUM
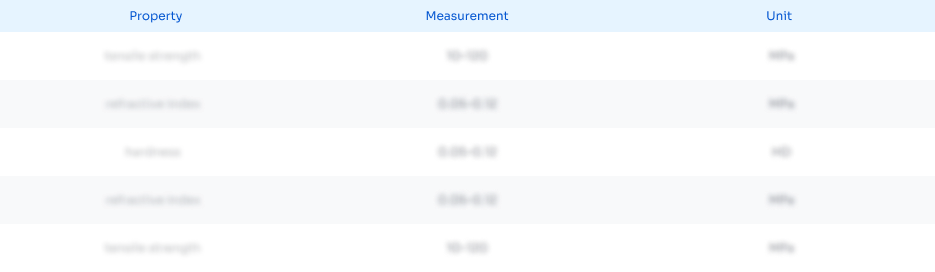
Abstract
Description
Claims
Application Information
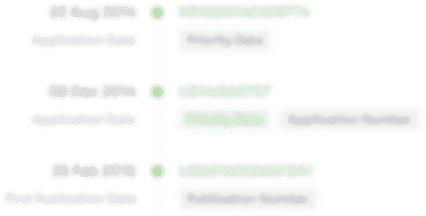
- R&D Engineer
- R&D Manager
- IP Professional
- Industry Leading Data Capabilities
- Powerful AI technology
- Patent DNA Extraction
Browse by: Latest US Patents, China's latest patents, Technical Efficacy Thesaurus, Application Domain, Technology Topic, Popular Technical Reports.
© 2024 PatSnap. All rights reserved.Legal|Privacy policy|Modern Slavery Act Transparency Statement|Sitemap|About US| Contact US: help@patsnap.com