Intelligent pruning method and system for deep network compression
A deep network and pruning technology, applied in the field of intelligent pruning methods and systems, can solve the problems of security check channel congestion, limited computing resources, complex network structure, etc., to avoid congestion, less computing resources, and avoid computing redundancy. Effect
- Summary
- Abstract
- Description
- Claims
- Application Information
AI Technical Summary
Problems solved by technology
Method used
Image
Examples
Embodiment 1
[0045] Convolutional neural network is a representative neural network in the field of deep learning technology, and it is also an important basis for the breakthrough achievements of deep learning technology in the field of computer vision. As the complexity of the task increases, the number of layers of the convolutional neural network is also increasing, and the scale of the model is also increasing. Today’s mainstream deep neural networks often have millions of parameters, making model training exponentially more difficult. In order to deploy trained deep convolutional neural networks on IoT and edge devices and achieve real-time fast inference, it is necessary to compress these deep convolutional neural networks to effectively reduce the memory space occupied by the model and the requirements for model inference. energy consumption.
[0046] Please refer to figure 1 and figure 2 , figure 1 is a schematic diagram of an intelligent pruning method for deep network compr...
Embodiment 2
[0088] This embodiment illustrates the effects of the intelligent pruning method and system for deep network compression of the present invention through a specific simulation experiment.
[0089] 1. Simulation conditions
[0090] In this example, the CPU is configured as Intel(R) Core(TM) i7-9700K CPU@3.60GHz, the memory is 32G, the GPU is a single-card NVIDIA GeForce RTX 2070 video memory, the CUDA version is 11.3, and the Windows 10 operating system is used on a PC. , using the Python language to complete the simulation experiment.
[0091] 2. Content of the simulation experiment
[0092] The data used in this embodiment is a total of 60,000 three-channel color images in 10 different categories of CIFAR10. The training data for each category is 5,000 images, and the test data is 1,000 images. When evaluating the importance of the filter, the number of divided intervals is N=10, the pruning ratio is T=0.6, the training optimizer uses mini-batch SGD, adds momentum and weigh...
PUM
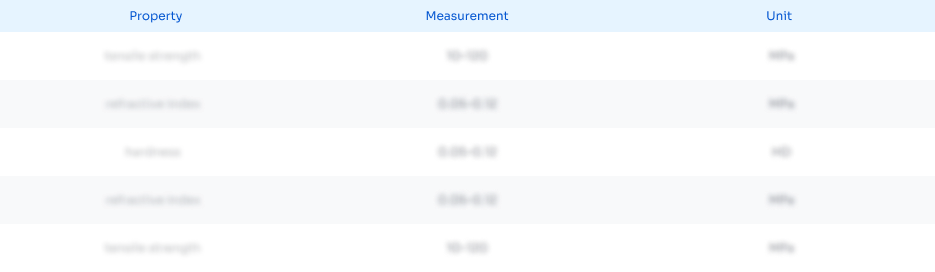
Abstract
Description
Claims
Application Information
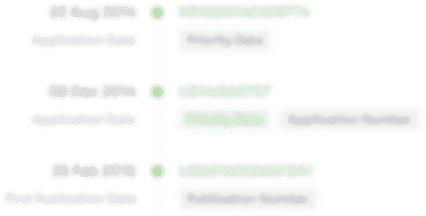
- R&D Engineer
- R&D Manager
- IP Professional
- Industry Leading Data Capabilities
- Powerful AI technology
- Patent DNA Extraction
Browse by: Latest US Patents, China's latest patents, Technical Efficacy Thesaurus, Application Domain, Technology Topic, Popular Technical Reports.
© 2024 PatSnap. All rights reserved.Legal|Privacy policy|Modern Slavery Act Transparency Statement|Sitemap|About US| Contact US: help@patsnap.com