Multi-modal cerebral apoplexy lesion segmentation method and system based on small sample learning
A multi-modal, small-sample technology, applied in neural learning methods, image analysis, biological neural network models, etc., can solve problems such as unclear boundaries, boundary restrictions, and different sizes of lesion information, to make up for insufficient data samples, The effect of improving accuracy
- Summary
- Abstract
- Description
- Claims
- Application Information
AI Technical Summary
Problems solved by technology
Method used
Image
Examples
Embodiment Construction
[0029] In order to make the objectives, technical solutions and advantages of the present invention clearer, the present invention will be further described in detail below with reference to the accompanying drawings.
[0030] like figure 1 , figure 2 As shown in the figure, the present invention first conducts image augmentation training for multi-modal images through generative confrontation, and then extracts different feature information in multiple modalities through multi-modal fusion, and finally puts the fused image into the Transformer. The semantic segmentation network segmented the image and finally obtained the prediction result.
[0031] Firstly, the data augmentation operation is performed on the multimodal image. Image data augmentation includes image deformation, Gaussian filtering and denoising, image scaling, and generation of adversarial image augmentation.
[0032] Among them such as image 3 The specific operation of the image augmentation of the gene...
PUM
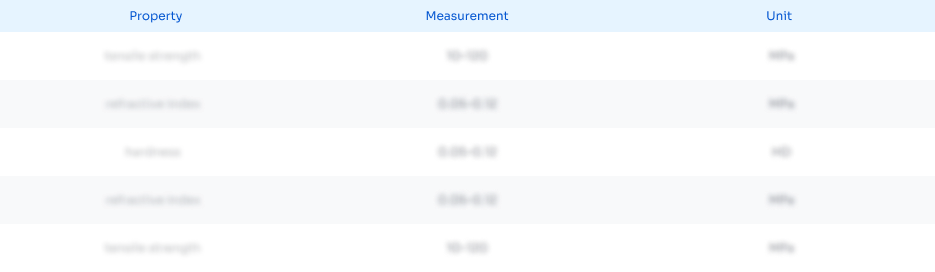
Abstract
Description
Claims
Application Information
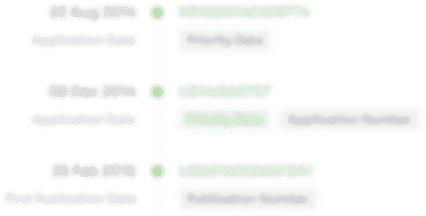
- R&D
- Intellectual Property
- Life Sciences
- Materials
- Tech Scout
- Unparalleled Data Quality
- Higher Quality Content
- 60% Fewer Hallucinations
Browse by: Latest US Patents, China's latest patents, Technical Efficacy Thesaurus, Application Domain, Technology Topic, Popular Technical Reports.
© 2025 PatSnap. All rights reserved.Legal|Privacy policy|Modern Slavery Act Transparency Statement|Sitemap|About US| Contact US: help@patsnap.com