Semi-supervised medical image segmentation method based on geometric consistency constraint
A medical image and image segmentation technology, applied in image analysis, neural learning methods, image data processing, etc., can solve the problems of not considering the difficulty of segmentation in different regions, not considering the quality of the original image data, affecting the segmentation performance, etc. , to achieve the effect of improving training efficiency, good generalization, and reducing uncertainty
- Summary
- Abstract
- Description
- Claims
- Application Information
AI Technical Summary
Problems solved by technology
Method used
Image
Examples
Embodiment Construction
[0042] The present invention will be described in further detail below in conjunction with the accompanying drawings and specific examples.
[0043] Medical image segmentation is an important part in the diagnosis and analysis of many diseases. In recent years, with the rapid development of imaging technology, the number of medical images has increased exponentially. The study of efficient and accurate automatic segmentation algorithms for medical images is of great significance for promoting the development of medical research and human health. However, the data quality of medical images is often uneven and the target boundary information is complex, such as figure 1 As shown, the usual segmentation results have high uncertainty. The present invention fuses the geometric structure information of the segmentation target, and achieves better results in the accuracy and completeness of the segmentation. The following takes the public dataset of the 2018 MICCAI Left Atrial Segm...
PUM
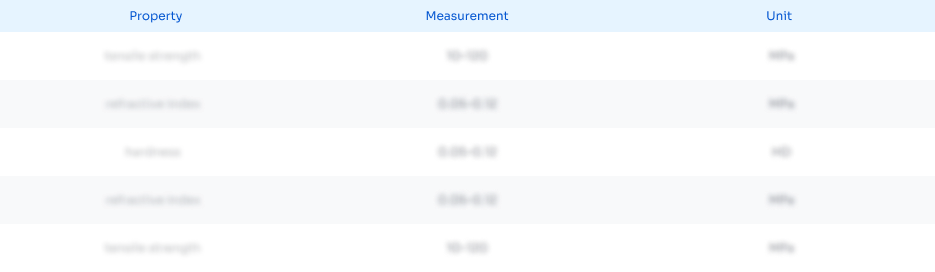
Abstract
Description
Claims
Application Information
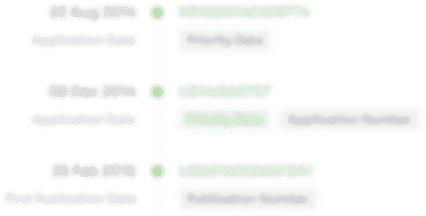
- R&D
- Intellectual Property
- Life Sciences
- Materials
- Tech Scout
- Unparalleled Data Quality
- Higher Quality Content
- 60% Fewer Hallucinations
Browse by: Latest US Patents, China's latest patents, Technical Efficacy Thesaurus, Application Domain, Technology Topic, Popular Technical Reports.
© 2025 PatSnap. All rights reserved.Legal|Privacy policy|Modern Slavery Act Transparency Statement|Sitemap|About US| Contact US: help@patsnap.com