Neural network modeling method and system
A neural network modeling and neural network model technology, applied in the field of neural network modeling methods and systems, can solve problems such as slow convergence speed, increased computational complexity of optimization problems, and difficulties in the optimization process, to achieve enhanced reliability and enhanced learning Ability and generalization ability, avoiding the effect of precision loss problem
- Summary
- Abstract
- Description
- Claims
- Application Information
AI Technical Summary
Problems solved by technology
Method used
Image
Examples
Embodiment Construction
[0033] As mentioned above, it is of great significance to obtain the neural network model whose input-output relationship (ie gain) is consistent with the actual production experience, to improve the reliability of the neural network prediction model and to promote the application of neural network in actual industrial production.
[0034] The present invention proposes a neural network modeling method to characterize the relationship between input and output, see figure 1 , is a flow chart of the method of the present invention, including:
[0035] S101: Step of data preprocessing: collect historical data of the actual process, and filter out sample data;
[0036] S102: the step of initializing the neural network: initializing the parameters of the neural network model;
[0037] S103: step of output calculation: calculate the output of the neural network model according to the model and sample input;
[0038] S104: Step of gain calculation: calculate the gain of model outpu...
PUM
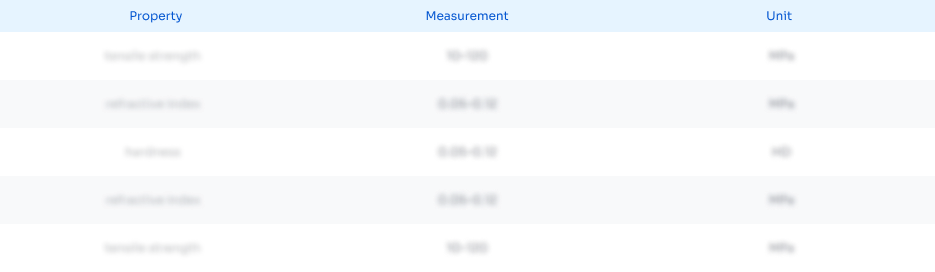
Abstract
Description
Claims
Application Information
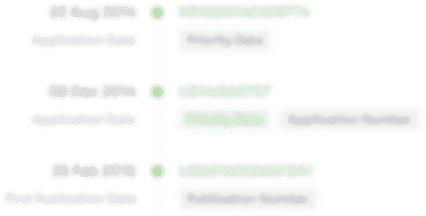
- R&D Engineer
- R&D Manager
- IP Professional
- Industry Leading Data Capabilities
- Powerful AI technology
- Patent DNA Extraction
Browse by: Latest US Patents, China's latest patents, Technical Efficacy Thesaurus, Application Domain, Technology Topic, Popular Technical Reports.
© 2024 PatSnap. All rights reserved.Legal|Privacy policy|Modern Slavery Act Transparency Statement|Sitemap|About US| Contact US: help@patsnap.com