Four-classifier cooperative training method combining active learning
A technology of active learning and collaborative training, applied in the fields of instruments, character and pattern recognition, computer parts, etc., it can solve the problems of waste of unlabeled samples, inconsistent determination of unlabeled sample categories, and enlargement, so as to improve the accuracy rate and increase the Effects and conditions that require high effects
- Summary
- Abstract
- Description
- Claims
- Application Information
AI Technical Summary
Problems solved by technology
Method used
Image
Examples
Embodiment 1
[0035] The present invention is a four-classifier collaborative training method combined with active learning, that is, the CTA method. Taking iris as an example, the implementation process of the CTA method is as follows:
[0036] Input: an unlabeled dataset D containing 96 samples u , a labeled dataset D containing 24 samples 1 , a test set T containing 30 samples.
[0037] Output: Classification error rate on the test set T.
[0038] ① Select the naive Bayesian algorithm L that is sensitive to the data set;
[0039] ② For the unlabeled data set D containing 96 samples taken out u , a labeled data set (initial training set) D containing 24 samples 1 , a test set T containing 30 samples and a naive Bayesian algorithm L, combined with figure 1 to D 1 Using Bootstrap technology to extract four times, the number of samples obtained is equal to |D 1 |The four training sets S 1 , S 2 , S 3 , S 4 , use the algorithm L to train the classifier C 1 , C 2 , C 3 , C 4 ; ...
Embodiment 2
[0050] The cooperative training method of four classifiers combined with active learning is the same as in Embodiment 1, taking thyroid as an example, see image 3 , the specific process is as follows:
[0051] Take out 552 data into the marked data set, take out 138 data into the unmarked data set, and put the remaining data into the test set. On the basis of the labeled data set, four training sample sets with a size of 552 are taken out by the Boostrap method. The four training sample sets are trained separately with the selected learning algorithm, and four classifiers are obtained. Use these four classifiers to determine the data in the unlabeled data set. For a classifier C, if the judgment results of the other three classifiers are the same, then this data is marked as the judgment result of the classifier, and then added to In the training sample set corresponding to classifier C, if the judgment results of the other three classifiers are different from each other, t...
Embodiment 3
[0054] The cooperative training method of four classifiers combined with active learning is the same as in Example 1-2, taking wine as an example, the specific implementation method is as in Example 1, and verified by experiments, such as figure 2 The results of the wine data set shown, the learning effect of the CTA method is better than the Co-Training method and the Tri-Training method; in the 10 experiments of CTA, the number of unlabeled samples is 114, and the average number of active learning is 1.7 times, indicating that The invention uses as few queries as possible to obtain strong generalization ability, and is a semi-supervised learning method with simple implementation, higher recognition rate and good effect.
PUM
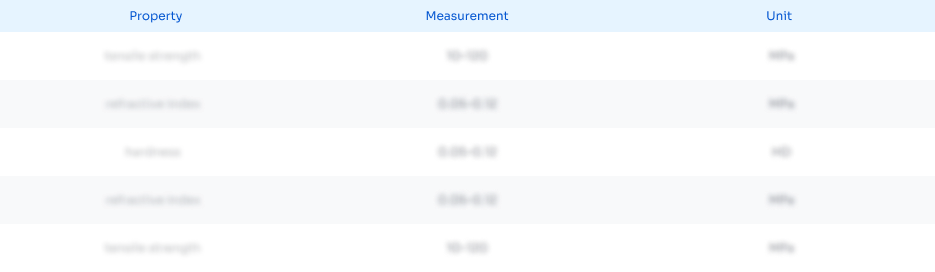
Abstract
Description
Claims
Application Information
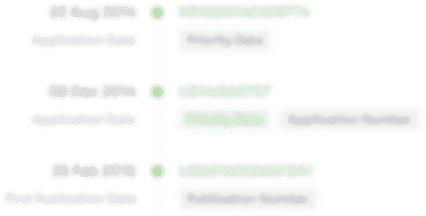
- R&D Engineer
- R&D Manager
- IP Professional
- Industry Leading Data Capabilities
- Powerful AI technology
- Patent DNA Extraction
Browse by: Latest US Patents, China's latest patents, Technical Efficacy Thesaurus, Application Domain, Technology Topic, Popular Technical Reports.
© 2024 PatSnap. All rights reserved.Legal|Privacy policy|Modern Slavery Act Transparency Statement|Sitemap|About US| Contact US: help@patsnap.com