Wavelet weighted multi-modulus blind equalization algorithm based on fractional lower order statistics (WT-FLOSWMMA)
A multi-mode blind equalization, fractional low-order technology, used in baseband system components, multi-carrier systems, shaping networks in transmitters/receivers, etc.
- Summary
- Abstract
- Description
- Claims
- Application Information
AI Technical Summary
Problems solved by technology
Method used
Image
Examples
Embodiment
[0102] [Example 1] The underwater acoustic channel c=[0.3132, -0.1040, 0.8908, 0.3134], and the transmission sequence is 64QAM. The signal-to-noise ratio is 25dB α-stable noise, its characteristic index α=1.7, β″=b=0, γ is determined by the signal-to-noise ratio SNR, γ=σ 2 / 10 SNR / 10 (σ 2 is the variance of the input sequence). In FLOSCMA, the step size factor μ 1 = 0.00008; in FLOSWMMA, the step factor μ 2 =0.00008; in the WT-FLOSWMMA of the present invention, the step size factor μ 3 =0.005, the length of the equalizer is 16, the fifth tap coefficient is initialized to 1, and the rest are all 0, the weighting factors are all λ=1.7, using db2 wavelet, second-order decomposition, the power is initialized to 10, smoothing factor β'= 0.99. The simulation results of Monte Carlo 3000 times, such as figure 2 shown.
[0103] from figure 2 (d) It can be seen that in the alpha stable noise environment, the convergence rate of WT-FLOSWMMA of the present invention is about 10...
Embodiment 2
[0105] [Example 2] Channel c=[0.9656, -0.0906, 0.0578, 0.2368], and the transmission sequence is 256QAM. α-stable noise with a signal-to-noise ratio of 30dB, in FLOSCMA, the step factor μ 1 = 0.00001; in FLOSWMMA, the step factor μ 2 =0.00002, weighting factor λ 1 =1.7; In the WT-FLOSWMMA of the present invention, the step size factor μ 3 =0.009, weighting factor λ 2 =1.8, the equalizer length is 16, the 8th tap coefficient is initialized to 1, all the other are 0, other parameters are all the same as in embodiment 1, the simulation result of Monte Carlo 4000 times, such as image 3 shown.
[0106] from image 3 (d) It can be seen that the steady-state error of WT-FLOSWMMA of the present invention is about 1dB smaller than FLOSWMMA, and 7dB smaller than FLOSCMA; the convergence speed of WT-FLOSWMMA of the present invention is about 2000 steps faster than FLOSWMMA, and about 6000 steps faster than FLOSCMA. And the output signal constellation diagram of the WT-FLOSWMMA of ...
PUM
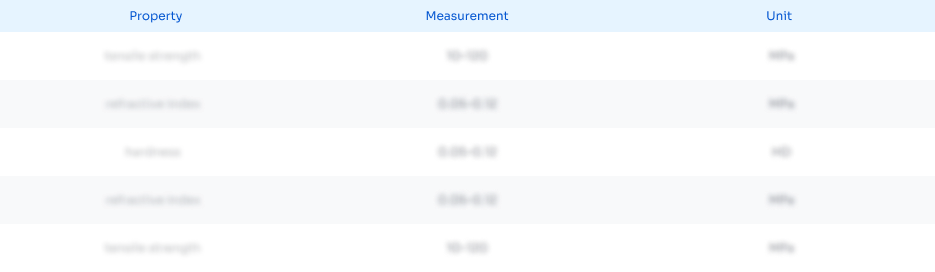
Abstract
Description
Claims
Application Information
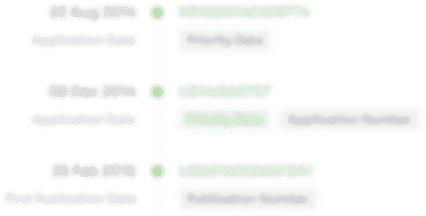
- R&D Engineer
- R&D Manager
- IP Professional
- Industry Leading Data Capabilities
- Powerful AI technology
- Patent DNA Extraction
Browse by: Latest US Patents, China's latest patents, Technical Efficacy Thesaurus, Application Domain, Technology Topic, Popular Technical Reports.
© 2024 PatSnap. All rights reserved.Legal|Privacy policy|Modern Slavery Act Transparency Statement|Sitemap|About US| Contact US: help@patsnap.com