Hyper spectral image classification method based on wavelet threshold denoising and empirical mode decomposition
An empirical mode decomposition and hyperspectral image technology, applied in the field of remote sensing, can solve the problems of insufficient classification accuracy and large wavelet noise, and achieve the effect of fast classification, good separability, and improved accuracy.
- Summary
- Abstract
- Description
- Claims
- Application Information
AI Technical Summary
Problems solved by technology
Method used
Image
Examples
specific Embodiment approach 1
[0025] Specific implementation mode 1. Combination figure 1 Describe this embodiment, a hyperspectral image classification method based on wavelet threshold noise reduction and empirical mode decomposition, the specific method is:
[0026] Step 1, two-dimensional wavelet threshold denoising of the hyperspectral image;
[0027] Step 2, performing empirical mode decomposition and image reconstruction on the hyperspectral image after wavelet noise reduction;
[0028] Step 3: Use the SVM classifier to classify the hyperspectral reconstructed image nIMFs to obtain classification accuracy.
specific Embodiment approach 2
[0029] Specific embodiment two, combine figure 2 This embodiment is described. The difference between this embodiment and the first embodiment is that the specific method of the two-dimensional wavelet threshold denoising of the hyperspectral image in the first step is:
[0030] Step 1.1. Input the hyperspectral data, and normalize the hyperspectral data to obtain the grayscale image x; select the wavelet decomposition layer number i=1, and perform the original signal x i Perform wavelet decomposition to obtain wavelet coefficient d, namely:
[0031] d=DWT{x}
[0032] Step 1.2. Calculate x i The wavelet threshold value of , and threshold the wavelet coefficients, namely:
[0033] d' = η τ (d)
[0034] Among them, η τ () is a threshold processing function with a threshold value of τ, using soft threshold denoising, as shown in the following formula:
[0035] d ′ = η τ ( ...
specific Embodiment approach 3
[0039] Specific embodiment three, combine image 3 , Figure 5 , Figure 6 , Figure 7 , Figure 8 , Figure 9 , Figure 10 , Figure 11 , Figure 12 , Figure 13 , Figure 14 and Figure 15 Describe this embodiment, the difference between this embodiment and specific embodiment 1 is that in step 2, the specific method for empirical mode decomposition and image reconstruction of the hyperspectral image after wavelet noise reduction is:
[0040] Step 2.1. Perform empirical mode decomposition on the hyperspectral image after wavelet noise reduction, and decompose it into q internal solid mode functions IMF and a residual:
[0041] Suppose X' k is the kth band image of the filtered hyperspectral image, x′(m,n) is the image X′ k Values at pixel points (m, n) (m=1, 2,..., M; n=1, 2,..., N); r ij (m, n) is used to calculate the i (i=1, 2, ..., I) IMF process, the input value of the j (j = 1, 2, ..., J) iteration, the kth Band image X' k As an input signal, the origina...
PUM
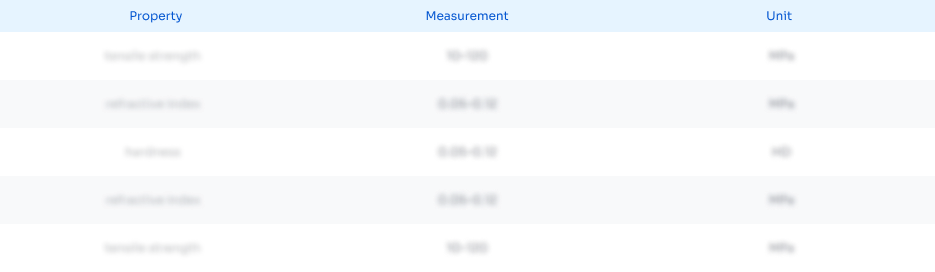
Abstract
Description
Claims
Application Information
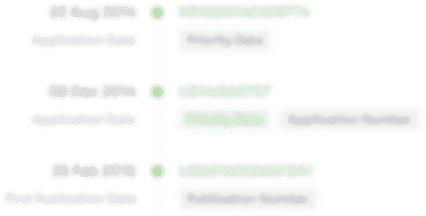
- R&D Engineer
- R&D Manager
- IP Professional
- Industry Leading Data Capabilities
- Powerful AI technology
- Patent DNA Extraction
Browse by: Latest US Patents, China's latest patents, Technical Efficacy Thesaurus, Application Domain, Technology Topic, Popular Technical Reports.
© 2024 PatSnap. All rights reserved.Legal|Privacy policy|Modern Slavery Act Transparency Statement|Sitemap|About US| Contact US: help@patsnap.com