Second-generation wavelet electromyographic signal noise eliminating method based on ensemble empirical mode decomposition
An empirical mode decomposition, electromyographic signal technology, applied in medical science, sensors, diagnostic recording/measurement, etc., can solve the problems of mode confusion, lack of adaptability, stationarity assumption, etc.
- Summary
- Abstract
- Description
- Claims
- Application Information
AI Technical Summary
Problems solved by technology
Method used
Image
Examples
Embodiment Construction
[0045] The embodiments of the present invention are described in detail below in conjunction with the accompanying drawings: this embodiment is implemented on the premise of the technical solution of the present invention, and detailed implementation methods and specific operating procedures are provided, but the protection scope of the present invention is not limited to the following the described embodiment.
[0046] Such as figure 1 As shown, this embodiment includes the following steps:
[0047] Step 1, in the noisy original EMG signal White noise with zero mean and constant standard deviation is added to , which is
[0048] (1)
[0049] In the formula, is the noise-containing original EMG signal of this embodiment, such as figure 2 shown, the signal length =2500, for the first The signal after adding white noise for the second time, for the first The white noise added for the first time, the standard deviation of the added...
PUM
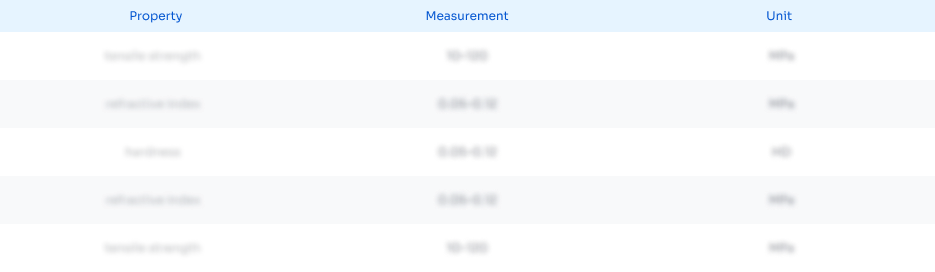
Abstract
Description
Claims
Application Information
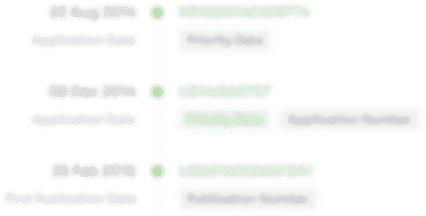
- R&D Engineer
- R&D Manager
- IP Professional
- Industry Leading Data Capabilities
- Powerful AI technology
- Patent DNA Extraction
Browse by: Latest US Patents, China's latest patents, Technical Efficacy Thesaurus, Application Domain, Technology Topic, Popular Technical Reports.
© 2024 PatSnap. All rights reserved.Legal|Privacy policy|Modern Slavery Act Transparency Statement|Sitemap|About US| Contact US: help@patsnap.com