Super-resolution image reconstruction method based on structure self-similarity and sparse representation
A super-resolution reconstruction and high-resolution image technology, which is applied in the field of image processing, can solve problems such as large amount of calculation, general image quality, and long time consumption, and achieve the effect of improving quality
- Summary
- Abstract
- Description
- Claims
- Application Information
AI Technical Summary
Problems solved by technology
Method used
Image
Examples
Embodiment 1
[0053] The present invention is an image super-resolution reconstruction method based on structural self-similarity and sparse representation. figure 1 , concrete steps of the present invention include:
[0054] Step 1. Input the training sample image pair, and use the training sample image pair to learn to construct a pair of low-resolution dictionaries D with a size of K l and the corresponding high-resolution dictionary D h , to construct a pair of low-resolution dictionaries D of size K l and the corresponding high-resolution dictionary D h The process includes:
[0055] 1a) Input the training image pair, and filter the low-resolution image to extract features. The filter used is f 1 =[-1,0,1], f 3 =[1,0,-2,0,1], The training images used are standard natural images commonly used in the field of image processing. These images can be selected from the library of classical methods, see Figure 2-Figure 5 ,in, figure 2 is the plant training image used in the presen...
Embodiment 2
[0076] The image super-resolution reconstruction method based on structural self-similarity and sparse representation is the same as that in Embodiment 1.
[0077] Wherein, the process of obtaining the weight matrix W in the objective function in step 4.2 includes:
[0078] 4.2a) The jth image block x in the image X to be corrected j In the neighbor area of , find the image block x j The kth nearest neighbor block x k , image block x j The indexes of all the neighbor blocks form the neighbor index set N(j);
[0079] 4.2b) Calculate the image block x according to the following formula j and its neighbor block x k The similarity weight w(j,k) of :
[0080] w ( j , k ) = exp { - | | x j ...
Embodiment 3
[0101] The image super-resolution reconstruction method based on structural self-similarity and sparse representation is the same as in Embodiment 1-2
[0102] 1) Experimental conditions
[0103] The software MATLAB7.9.0 is used as the simulation tool, and the computer configuration is Intel Core2 / 1.8G / 2G.
[0104] 2) Experimental content
[0105] against Figure 6 (a) The displayed low-resolution Lena image is reconstructed by Bicubic interpolation method, Yang (TIP2010) method and the present invention. Obtain the reconstructed image results of the respective methods, and the reconstruction results of the Bicubic interpolation method can be found in Figure 6 (b), the reconstruction results of Yang (TIP2010) method are shown in Figure 6 (c), the super-resolution reconstruction results of the present invention are shown in Figure 6 (d).
[0106] The present invention only reconstructs the grayscale component of the input image, that is, in step 2, the low-resolution L...
PUM
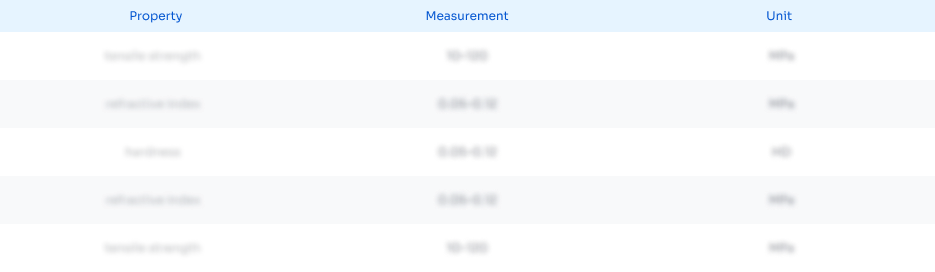
Abstract
Description
Claims
Application Information
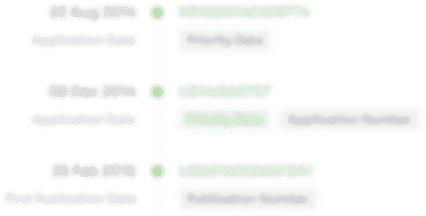
- R&D Engineer
- R&D Manager
- IP Professional
- Industry Leading Data Capabilities
- Powerful AI technology
- Patent DNA Extraction
Browse by: Latest US Patents, China's latest patents, Technical Efficacy Thesaurus, Application Domain, Technology Topic, Popular Technical Reports.
© 2024 PatSnap. All rights reserved.Legal|Privacy policy|Modern Slavery Act Transparency Statement|Sitemap|About US| Contact US: help@patsnap.com