Automatic sleep stage method based on electroencephalogram, heart rate variability and coherence between electroencephalogram and heart rate variability
A technology of heart rate variation and sleep staging, which is applied in the measurement of pulse rate/heart rate, medical science, sensors, etc. It can solve problems such as heavy workload, discomfort of subjects, and large disturbance of normal sleep, so as to improve accuracy and simplicity sex, considerable social and economic benefits
- Summary
- Abstract
- Description
- Claims
- Application Information
AI Technical Summary
Problems solved by technology
Method used
Image
Examples
Embodiment Construction
[0029] In order to make the object, technical solution and advantages of the present invention clearer, the implementation manner of the present invention will be further described in detail below in conjunction with the accompanying drawings.
[0030] As a physiological state, sleep must be reflected in multiple parts of the body and multiple physiological processes. EEG is a central nervous system signal, the most sensitive and accurate physiological signal for sleep staging, ECG signal is an autonomic nervous signal, and the extracted heart rate variability signal (HRV) reflects the activity level of the sympathetic nervous system and the parasympathetic nervous system, while the autonomic nervous system Regulatory ability varies with sleep levels. Studies have shown that autonomic nervous activity and sleep have a common regulatory center in the subcortex. The effective fusion of ECG signals as the main autonomic nervous signals and EEG signals is bound to improve the accu...
PUM
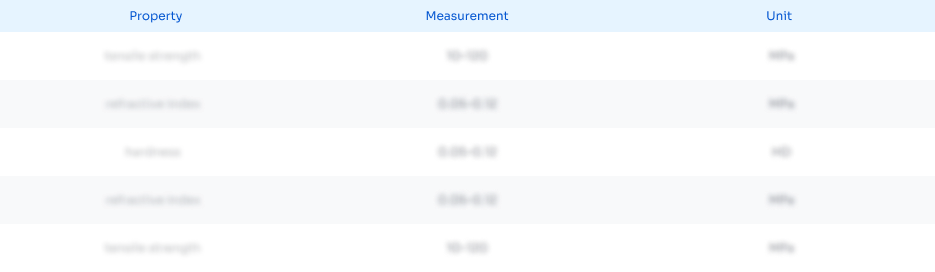
Abstract
Description
Claims
Application Information
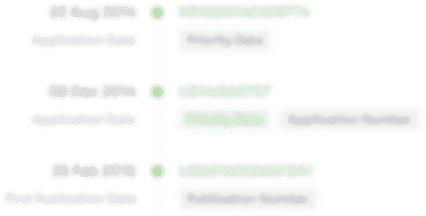
- R&D
- Intellectual Property
- Life Sciences
- Materials
- Tech Scout
- Unparalleled Data Quality
- Higher Quality Content
- 60% Fewer Hallucinations
Browse by: Latest US Patents, China's latest patents, Technical Efficacy Thesaurus, Application Domain, Technology Topic, Popular Technical Reports.
© 2025 PatSnap. All rights reserved.Legal|Privacy policy|Modern Slavery Act Transparency Statement|Sitemap|About US| Contact US: help@patsnap.com