PM2.5 concentration prediction method based on feature vectors and least square support vector machine
A support vector machine and eigenvector technology, applied in special data processing applications, instruments, electrical digital data processing, etc.
- Summary
- Abstract
- Description
- Claims
- Application Information
AI Technical Summary
Problems solved by technology
Method used
Image
Examples
Embodiment 1
[0050] Embodiment 1: as Figure 1-4 As shown, a PM2.5 concentration prediction method based on eigenvectors and least squares support vector machine, first collects the pollutant concentration data related to PM2.5 concentration for preprocessing; then calculates the comprehensive meteorological index; .5 Concentration-related pollutant concentration data and comprehensive meteorological index are carried out for correlation analysis, and the eigenvectors containing the comprehensive meteorological index are obtained to form the eigenvector A and the eigenvectors obtained by removing the comprehensive meteorological index are used to form the eigenvector B; finally, through the eigenvector A, The feature vector B constitutes the training sample to train the LS-SVM model and evaluate the prediction results.
[0051] The concrete steps of described method are as follows:
[0052] Step1. Collect the pollutant concentration data related to PM2.5 concentration for preprocessing: s...
Embodiment 2
[0067] Embodiment 2: as Figure 1-4 As shown, a PM2.5 concentration prediction method based on eigenvectors and least squares support vector machine, first collects the pollutant concentration data related to PM2.5 concentration for preprocessing; then calculates the comprehensive meteorological index; .5 Concentration-related pollutant concentration data and comprehensive meteorological index are carried out for correlation analysis, and the eigenvectors containing the comprehensive meteorological index are obtained to form the eigenvector A and the eigenvectors obtained by removing the comprehensive meteorological index are used to form the eigenvector B; finally, through the eigenvector A, The feature vector B constitutes the training sample to train the LS-SVM model and evaluate the prediction results.
[0068] The concrete steps of described method are as follows:
[0069] Step1. Collect the pollutant concentration data related to PM2.5 concentration for preprocessing: s...
Embodiment 3
[0084] Embodiment 3: as Figure 1-4 As shown, a PM2.5 concentration prediction method based on eigenvectors and least squares support vector machine, first collects the pollutant concentration data related to PM2.5 concentration for preprocessing; then calculates the comprehensive meteorological index; .5 Concentration-related pollutant concentration data and comprehensive meteorological index are carried out for correlation analysis, and the eigenvectors containing the comprehensive meteorological index are obtained to form the eigenvector A and the eigenvectors obtained by removing the comprehensive meteorological index are used to form the eigenvector B; finally, through the eigenvector A, The feature vector B constitutes the training sample to train the LS-SVM model and evaluate the prediction results.
[0085] The concrete steps of described method are as follows:
[0086] Step 1. Collect the pollutant concentration data related to PM2.5 concentration for preprocessing: ...
PUM
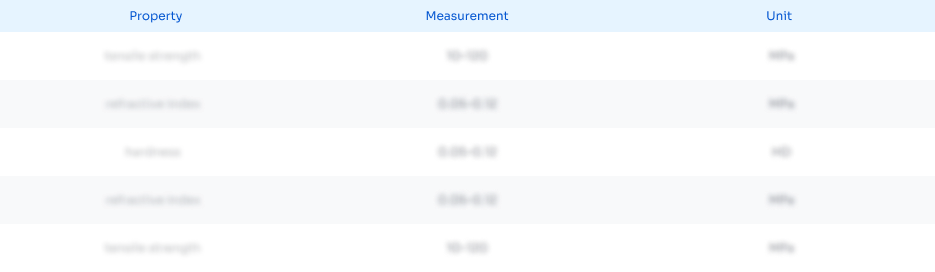
Abstract
Description
Claims
Application Information
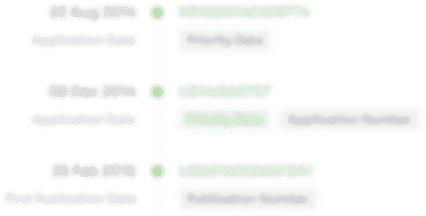
- R&D Engineer
- R&D Manager
- IP Professional
- Industry Leading Data Capabilities
- Powerful AI technology
- Patent DNA Extraction
Browse by: Latest US Patents, China's latest patents, Technical Efficacy Thesaurus, Application Domain, Technology Topic.
© 2024 PatSnap. All rights reserved.Legal|Privacy policy|Modern Slavery Act Transparency Statement|Sitemap