Extracting method of optical spectrum vector cross-correlation features in hyper-spectral image classification
A hyperspectral image and spectral vector technology, which is applied in the field of extraction of spectral vector cross-correlation features in hyperspectral image classification, can solve the problems of increasing the difficulty of fine classification, high dependence on spectral feature databases, and large data redundancy.
- Summary
- Abstract
- Description
- Claims
- Application Information
AI Technical Summary
Problems solved by technology
Method used
Image
Examples
Embodiment Construction
[0073] The present invention will be further described below in conjunction with the accompanying drawings and embodiments.
[0074] like figure 1 As shown, the process of the autocorrelation feature extraction method is:
[0075] 1) Data conversion. Transform the original 3D hyperspectral data I into 2D feature data I 1 , each row corresponds to a sample, and each column corresponds to a feature.
[0076] 2) Data normalization. Data normalization completes a data mapping process. Step 1) I 1 The data is projected onto the interval [-1,1]. The procedure is to search for the minimum value x of the eigenvalues in each column min , the maximum value x max , will [x min ,x max ] is mapped to [-1,1].
[0077] 3) The principal component analysis method (PCA) is used to reduce the dimensionality and denoise of the data. On the basis of 2), data dimensionality reduction is required to represent as much original image information as possible with as few dimensions as poss...
PUM
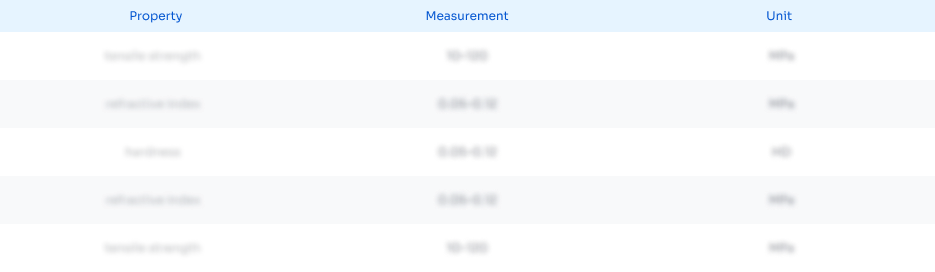
Abstract
Description
Claims
Application Information
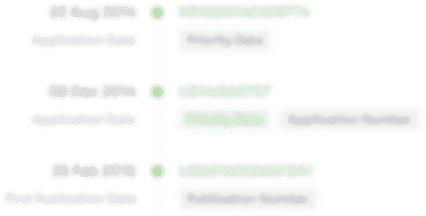
- R&D Engineer
- R&D Manager
- IP Professional
- Industry Leading Data Capabilities
- Powerful AI technology
- Patent DNA Extraction
Browse by: Latest US Patents, China's latest patents, Technical Efficacy Thesaurus, Application Domain, Technology Topic, Popular Technical Reports.
© 2024 PatSnap. All rights reserved.Legal|Privacy policy|Modern Slavery Act Transparency Statement|Sitemap|About US| Contact US: help@patsnap.com