GPU cluster-based multidimensional big data factorization method
A GPU cluster and big data technology, applied in the field of signal analysis, can solve problems such as result differentiation, high computational complexity, and insufficient computational resources
- Summary
- Abstract
- Description
- Claims
- Application Information
AI Technical Summary
Problems solved by technology
Method used
Image
Examples
Embodiment Construction
[0075] In order to facilitate those of ordinary skill in the art to understand and implement the present invention, the present invention will be described in further detail below in conjunction with the accompanying drawings and embodiments. It should be understood that the implementation examples described here are only used to illustrate and explain the present invention, and are not intended to limit this invention.
[0076] please see figure 1 , the present invention aims at the problem that the traditional grid parallel factor analysis model (gridPARAFAC) cannot handle large-scale, high-dimensional multidimensional data analysis, and proposes an effective multi-mode decomposition method for multi-dimensional big data based on (graphics processing unit) GPU clusters , that is, the hierarchical parallel factor analysis (H-PARAFAC) framework, which is based on gridPARAFAC (grid parallel factor analysis), includes the process of integrating tensor subsets under a coarse-grai...
PUM
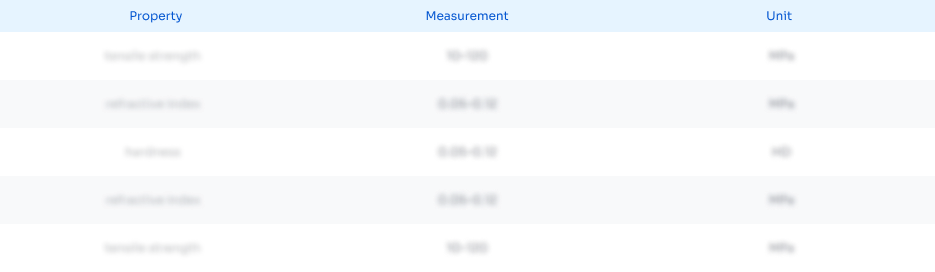
Abstract
Description
Claims
Application Information
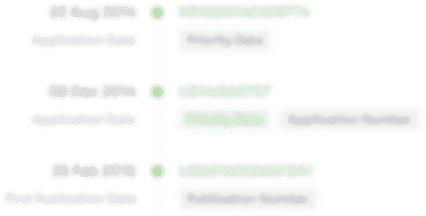
- R&D
- Intellectual Property
- Life Sciences
- Materials
- Tech Scout
- Unparalleled Data Quality
- Higher Quality Content
- 60% Fewer Hallucinations
Browse by: Latest US Patents, China's latest patents, Technical Efficacy Thesaurus, Application Domain, Technology Topic, Popular Technical Reports.
© 2025 PatSnap. All rights reserved.Legal|Privacy policy|Modern Slavery Act Transparency Statement|Sitemap|About US| Contact US: help@patsnap.com