Image structure-based particle swarm optimization non-convex compressed sensing image reconstruction method
A particle swarm optimization and compressed sensing technology, applied in the field of image processing, can solve the problems of slow reconstruction, unfavorable real-time applications, slow speed, etc., to reduce reconstruction time, shorten reconstruction time, and improve reconstruction accuracy.
- Summary
- Abstract
- Description
- Claims
- Application Information
AI Technical Summary
Problems solved by technology
Method used
Image
Examples
Embodiment Construction
[0024] Reference figure 1 , The implementation steps of the present invention are as follows:
[0025] Step 1. The compressed sensing receiver receives the observation vectors of all image blocks sent by the sender.
[0026] The sender inputs a 512×512 natural image and divides it into 16×16 non-overlapping blocks to obtain 1024 image blocks, and then performs Gaussian random observation on each image block to obtain the observation vector y of all image blocks 1 , Y 2 ,...,y n , And send the observation vector of all image blocks, where n represents the number of image blocks.
[0027] Step 2: Perform structure discrimination on the image block corresponding to each observation vector, mark the image block as an optical slider, a single direction block and a multi-direction block, and record the direction of the single direction block.
[0028] 2a) Calculate the variance of each observation vector and set the smoothing threshold to 0.45σ, where σ is the average of the variance of all ...
PUM
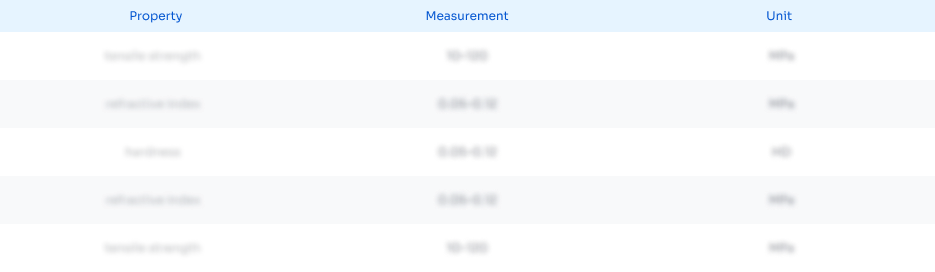
Abstract
Description
Claims
Application Information
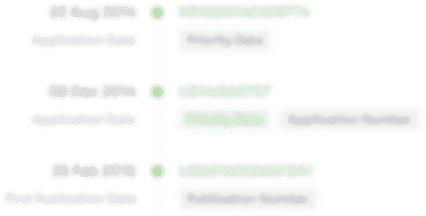
- R&D Engineer
- R&D Manager
- IP Professional
- Industry Leading Data Capabilities
- Powerful AI technology
- Patent DNA Extraction
Browse by: Latest US Patents, China's latest patents, Technical Efficacy Thesaurus, Application Domain, Technology Topic, Popular Technical Reports.
© 2024 PatSnap. All rights reserved.Legal|Privacy policy|Modern Slavery Act Transparency Statement|Sitemap|About US| Contact US: help@patsnap.com