Hyperspectral data classification method based on multi-layer convolution network and data organization and folding
A technology of data reorganization and convolutional network, applied in the field of hyperspectral pixel data classification, can solve the problems of increased processing complexity and differences in recognition results
- Summary
- Abstract
- Description
- Claims
- Application Information
AI Technical Summary
Problems solved by technology
Method used
Image
Examples
specific Embodiment approach 1
[0020] Specific implementation mode 1: This implementation mode provides a hyperspectral data classification method based on multi-layer convolutional network and data reorganization and folding. After the hyperspectral data is expanded and preprocessed, the feature dimension of the pixel spectral dimension is expanded. The three-dimensional data matrix is re-formed by folding with the spectral dimension, and the two-dimensional spectral feature map is obtained as the input of the classifier, and the convolutional neural network is constructed layer by layer. Perform deep feature extraction and network weight update, and finally make the connection weights and biases between layers converge to stability through multiple iterations. Finally, this network can be used to quickly and accurately classify unlabeled samples from the same data source.
[0021] The flow chart of the present invention is as figure 1 As shown, it is divided into four steps, and the specific steps are a...
specific Embodiment approach 2
[0063] Embodiment 2: In this embodiment, a hyperspectral data classification algorithm based on multi-layer convolutional network and data folding is applied to the KSC hyperspectral remote sensing data set. The KSC hyperspectral data set is a typical three-dimensional hyperspectral cube data with a size of 512×614×176. It was obtained by the National Aeronautics and Space Administration of the United States in 1996 by the AVIRIS sensor during an aviation flight at an altitude of about 20km. The hyperspectral The size of the remote sensing image is 614×512 pixels, the spatial resolution scale is 18m, and the collected spectral range is 400-2500nm. The image covers the ground object information near Florida Kennedy in the United States. After removing the bands affected by atmospheric absorption and noise, the available 176 bands. For the spectral sampling curves and corresponding labels of some pixel bands, see figure 2 .
[0064] Execute step 1: data loading and preprocess...
PUM
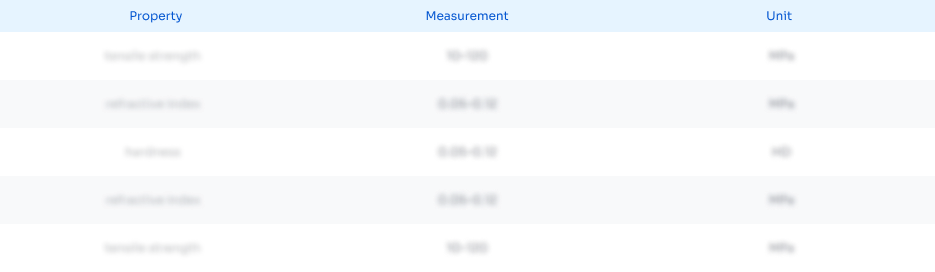
Abstract
Description
Claims
Application Information
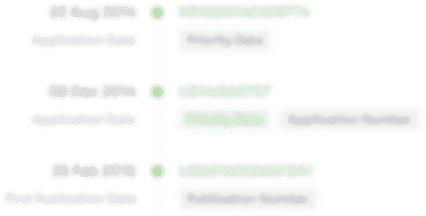
- R&D Engineer
- R&D Manager
- IP Professional
- Industry Leading Data Capabilities
- Powerful AI technology
- Patent DNA Extraction
Browse by: Latest US Patents, China's latest patents, Technical Efficacy Thesaurus, Application Domain, Technology Topic, Popular Technical Reports.
© 2024 PatSnap. All rights reserved.Legal|Privacy policy|Modern Slavery Act Transparency Statement|Sitemap|About US| Contact US: help@patsnap.com