An Online Fault Diagnosis Method Based on Massive Operating Data
A technology for fault diagnosis and operation data, applied in electrical digital data processing, special data processing applications, instruments, etc., can solve problems such as measurement value fluctuation, sample data imbalance, and harsh measurement environment, to ensure effectiveness and stability. , improve the diagnosis rate and fault tolerance, improve the effect of online monitoring accuracy
- Summary
- Abstract
- Description
- Claims
- Application Information
AI Technical Summary
Problems solved by technology
Method used
Image
Examples
Embodiment Construction
[0031] Below in conjunction with embodiment the present invention will be further described.
[0032] Such as figure 1 As shown, the online fault diagnosis method based on massive data includes the following steps: first determine the sample parameters of the fault diagnosis model, obtain the steady-state sample data, and eliminate redundant sample data; then use the optimal samples to train the fault diagnosis model; finally, in the real-time diagnosis process In the process, the monitoring data is standardized, and the unsteady data is eliminated from the processed data stream, and the trained fault diagnosis model is used to diagnose the sensor fault on the steady data, and the unsteady data is not used as the basis for judging the sensor fault , if there is fault data in the steady-state data sample, the fault diagnosis model will send out an alarm and handle the fault.
[0033]The entire online diagnosis process includes a model training module and an online steady-state...
PUM
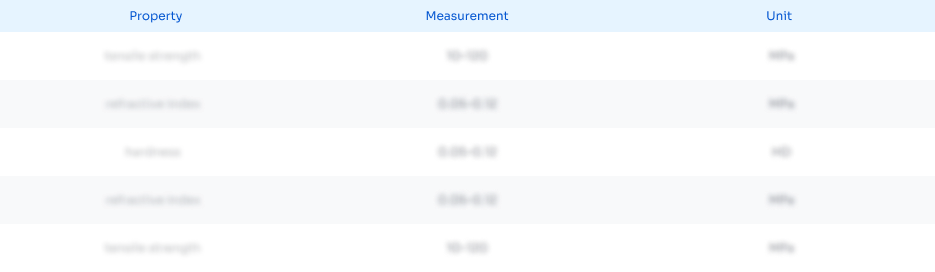
Abstract
Description
Claims
Application Information
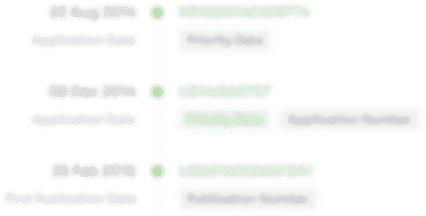
- R&D
- Intellectual Property
- Life Sciences
- Materials
- Tech Scout
- Unparalleled Data Quality
- Higher Quality Content
- 60% Fewer Hallucinations
Browse by: Latest US Patents, China's latest patents, Technical Efficacy Thesaurus, Application Domain, Technology Topic, Popular Technical Reports.
© 2025 PatSnap. All rights reserved.Legal|Privacy policy|Modern Slavery Act Transparency Statement|Sitemap|About US| Contact US: help@patsnap.com