Joint sparse representation and deep learning-based image super resolution method and system
A technology of sparse representation coefficients and deep learning, applied in the image super-resolution method and system field of joint sparse representation and deep learning, which can solve problems such as underfitting or overfitting
- Summary
- Abstract
- Description
- Claims
- Application Information
AI Technical Summary
Problems solved by technology
Method used
Image
Examples
Embodiment Construction
[0050] The present invention will be further described below in conjunction with the accompanying drawings and embodiments.
[0051] The embodiment of the present invention is super-resolution reconstruction of remote sensing image, refer to figure 1 , the concrete steps of the embodiment of the present invention are as follows:
[0052] Step a: Data generation
[0053] The present invention first cuts the high-resolution image in the training sample database into N d×d image blocks, reduces the resolution of each image block to obtain N corresponding low-resolution image blocks. Then the high-resolution image blocks are stretched into columns to form a matrix of column vectors (indicating y h for d 2 ×N real matrix), the same way to get the low-resolution image corresponding matrix Calculate the difference part y between the two hl =y h -y l .
[0054] Step b: training dictionary and corresponding sparse representation coefficients
[0055] will y l and y hl Per...
PUM
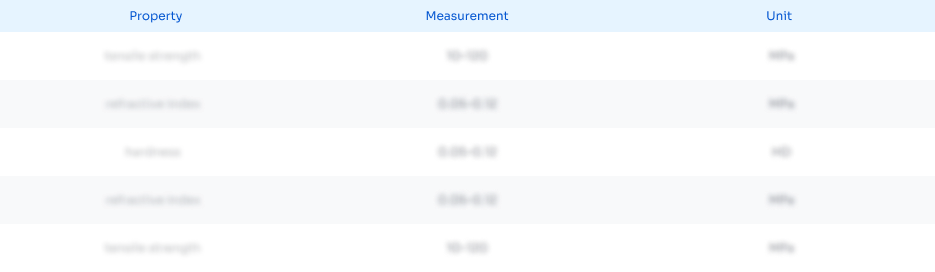
Abstract
Description
Claims
Application Information
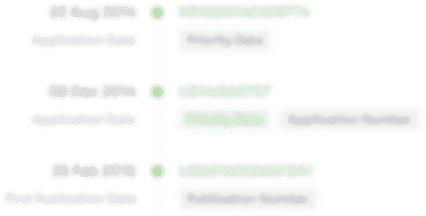
- R&D Engineer
- R&D Manager
- IP Professional
- Industry Leading Data Capabilities
- Powerful AI technology
- Patent DNA Extraction
Browse by: Latest US Patents, China's latest patents, Technical Efficacy Thesaurus, Application Domain, Technology Topic, Popular Technical Reports.
© 2024 PatSnap. All rights reserved.Legal|Privacy policy|Modern Slavery Act Transparency Statement|Sitemap|About US| Contact US: help@patsnap.com