Single-image super-resolution reconstruction algorithm based on optical flow method and sparse neighbor embedding
A super-resolution reconstruction and neighborhood embedding technology, which is applied in image analysis, image enhancement, image data processing, etc., can solve the problem that the low-frequency basic content interference cannot be fully eliminated, the matching accuracy cannot be greatly improved, and the Matching error minimization and other issues
- Summary
- Abstract
- Description
- Claims
- Application Information
AI Technical Summary
Problems solved by technology
Method used
Image
Examples
Embodiment Construction
[0059] Embodiments of the present invention will be described in detail below with reference to the accompanying drawings.
[0060] Such as figure 1 As shown, the present invention provides a single image super-resolution reconstruction algorithm based on optical flow method and sparse neighborhood embedding, including the following specific steps:
[0061] S1: Through offline dictionary training and learning, learn the corresponding features of high-resolution images and low-resolution images from the high-resolution images and corresponding low-resolution images in the prepared training image set, and use PCA dimensionality reduction algorithm and The k-means clustering method further processes the data collected in the learning process to form a dictionary. figure 2 It is the overall framework diagram of the dictionary learning and training process. in:
[0062] S11: Convert the training set image from the RGB color space to the YCbCr color space, and use its brightness...
PUM
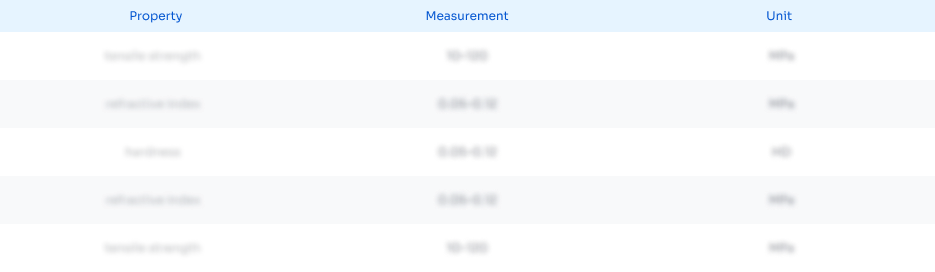
Abstract
Description
Claims
Application Information
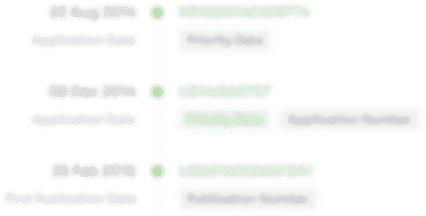
- R&D
- Intellectual Property
- Life Sciences
- Materials
- Tech Scout
- Unparalleled Data Quality
- Higher Quality Content
- 60% Fewer Hallucinations
Browse by: Latest US Patents, China's latest patents, Technical Efficacy Thesaurus, Application Domain, Technology Topic, Popular Technical Reports.
© 2025 PatSnap. All rights reserved.Legal|Privacy policy|Modern Slavery Act Transparency Statement|Sitemap|About US| Contact US: help@patsnap.com