Method for processing head structure image data based on deep learning algorithm
A technology of deep learning and head processing, which is applied in the field of medical image processing, can solve problems such as applied research and prediction, and achieve the effects of reducing work pressure, improving work efficiency and reducing work pressure
- Summary
- Abstract
- Description
- Claims
- Application Information
AI Technical Summary
Problems solved by technology
Method used
Image
Examples
Embodiment 1
[0037]The head magnetic resonance structural image data includes head structural magnetic resonance images of smokers and non-smokers, and the parameters of the head structural magnetic resonance images are: 3D T1-weighted MPRAGE T1 weighted sequence, sagittal scan, 176 layers , TR=2530ms, TE=3.39ms, slice thickness 1mm, TI=1100ms, FOV=256mm×256mm, resolution=256×256, repeated twice.
[0038] Such as figure 1 As shown, there are 176 MRI images of head structures in each case, and 1 / 4 of the smoking group and non-smoking group were randomly selected as the test set, including 15 smokers and 16 non-smokers, and the rest were used as the training set.
[0039] Efficiently handle image tasks through deep convolutional network models and recurrent neural networks (RNN). As a long-short-term memory recurrent neural network, LSTM can map variable-length inputs into variable-length outputs.
[0040] figure 2 shows the flowchart of the ConvLSTM model, as figure 2 As shown, firstl...
Embodiment 2
[0043] In Example 2, there are also 176 images of the MRI image of head structure in each case. The drug-taking group and the non-drug-taking group each randomly select 1 / 4 as the test set, including 15 cases of drug users, 16 cases of non-drug users, and the rest as the training set. .
[0044] All the other methods are the same as in Example 1.
Embodiment 3
[0046] In Example 3, there are also 176 images of head structure magnetic resonance images in each case, and each of the epileptic patient group and the non-epilepsy patient group randomly selects 1 / 4 as the test set, including 15 epileptic patients and 16 non-epilepsy patients. example, and the rest as the training set.
[0047] All the other methods are the same as in Example 1.
[0048] The method for processing head structure image data based on deep learning algorithm of the present invention uses LSTM model to analyze head structure image data, so as to better achieve classification effect.
PUM
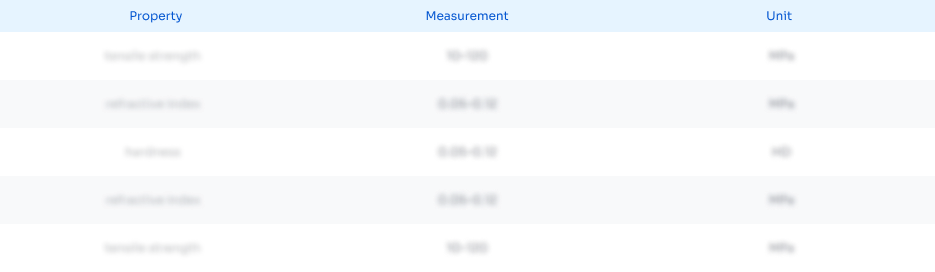
Abstract
Description
Claims
Application Information
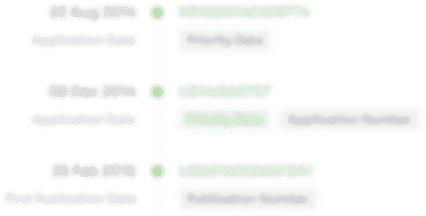
- R&D Engineer
- R&D Manager
- IP Professional
- Industry Leading Data Capabilities
- Powerful AI technology
- Patent DNA Extraction
Browse by: Latest US Patents, China's latest patents, Technical Efficacy Thesaurus, Application Domain, Technology Topic.
© 2024 PatSnap. All rights reserved.Legal|Privacy policy|Modern Slavery Act Transparency Statement|Sitemap