A concept connection method for medical diagnosis texts
A connection method and medical diagnosis technology, applied in the field of concept connection, can solve the problem of low recall rate and achieve the effect of easy transplantation and realization
- Summary
- Abstract
- Description
- Claims
- Application Information
AI Technical Summary
Problems solved by technology
Method used
Image
Examples
Embodiment Construction
[0019] The present invention will be described in detail below in conjunction with specific embodiments.
[0020] A concept linking method for medical diagnostic texts that utilizes recurrent neural networks (RNNs) to tackle the concept linking problem, with a particular focus on summarizing unseen concepts at test time with vectorized concept symbols, sharing their characteristics, and then Multiple layers predict the entire traversal, allowing the model to satisfactorily achieve good concatenation. The method includes the following steps, such as figure 2 as shown,
[0021] The first step 201 is to construct a recurrent neural network system (RNNs), such as figure 1 As shown, the components include:
[0022] A span encoder 101, which is a 2-layer 256-unit long-short-term memory encoder. The input span is represented by a word vector (pretrained by GloVe), and a 256-dimensional element embedding es is generated. The output corresponds to the previous time step hidden sta...
PUM
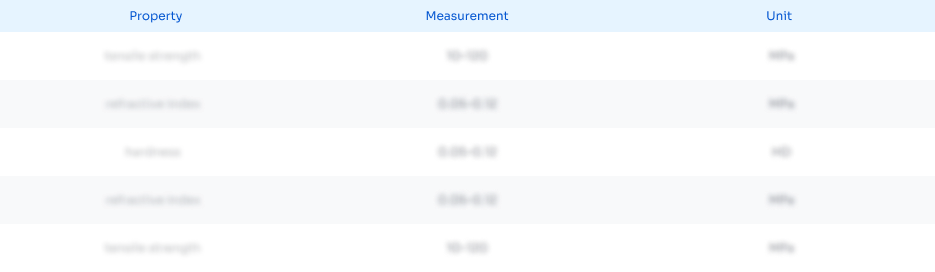
Abstract
Description
Claims
Application Information
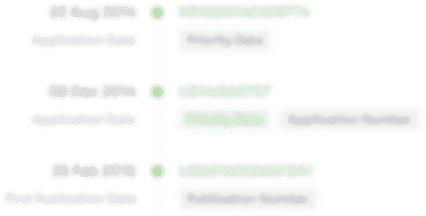
- Generate Ideas
- Intellectual Property
- Life Sciences
- Materials
- Tech Scout
- Unparalleled Data Quality
- Higher Quality Content
- 60% Fewer Hallucinations
Browse by: Latest US Patents, China's latest patents, Technical Efficacy Thesaurus, Application Domain, Technology Topic, Popular Technical Reports.
© 2025 PatSnap. All rights reserved.Legal|Privacy policy|Modern Slavery Act Transparency Statement|Sitemap|About US| Contact US: help@patsnap.com