Human behavior video identification method
A technology for video recognition and human body video, applied in the field of pattern recognition, it can solve problems such as poor results, no consideration of influence, and differences in clustering results.
- Summary
- Abstract
- Description
- Claims
- Application Information
AI Technical Summary
Problems solved by technology
Method used
Image
Examples
Embodiment 1
[0095] Embodiment 1 divides the joint points of the human body into five parts according to the joint point information of the human body skeleton; then obtains the energy characteristics of the human body behavior of each frame of image respectively. Finally, the human behavior action image sequence is clustered by SWAP to obtain the cluster center, which is the key frame of the image.
[0096] This embodiment includes the following steps:
[0097] Divide the 20 skeleton joint points of the human body into five parts of the human body:
[0098] f 1,t :Torso(J1, J2, J3, J4) F 2,t : Right upper limb (J5, J6, J7, J8)
[0099] f 3,t : Left upper limb (J9, J10, J11, J12) F 4,t : Right lower limb (J13, J14, J15, J16)
[0100] f 5,t : Left lower limb (J17, J18, J19, J20), see for details figure 2 .
[0101] 2. Calculate the kinetic energy and potential energy of the five parts separately, and obtain the 10-dimensional human behavior energy features, each feature represents...
Embodiment 2
[0114] Embodiment 2 is as table 1, image 3 Shown is the key frame extraction result of the embodiment. Table 1 illustrates the number of key frames extracted from the drinking action. As can be seen from Table 1, this embodiment extracts 37 key frames for the drinking action containing 1583 frames of images. image 3 is the key frame extraction result, because the drinking action is a cyclic action, including multiple drinking actions, so image 3 It shows the key frame extraction results of a cycle of the drinking action, which contains 9 key frames.
[0115] Table 1 Description of Human Behavior Library CAD-60
[0116]
[0117] In this embodiment, six actions in CAD-60 (cutting vegetables, drinking water, rinsing mouth, standing, making a phone call, and writing on a blackboard) are used to illustrate that the present invention can improve the speed of human behavior recognition. Through the steps of the embodiment, key frames can be extracted for six actions, and ...
PUM
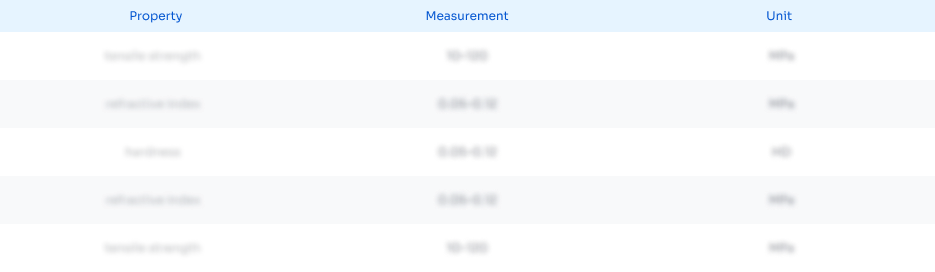
Abstract
Description
Claims
Application Information
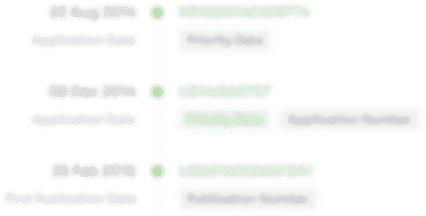
- R&D Engineer
- R&D Manager
- IP Professional
- Industry Leading Data Capabilities
- Powerful AI technology
- Patent DNA Extraction
Browse by: Latest US Patents, China's latest patents, Technical Efficacy Thesaurus, Application Domain, Technology Topic, Popular Technical Reports.
© 2024 PatSnap. All rights reserved.Legal|Privacy policy|Modern Slavery Act Transparency Statement|Sitemap|About US| Contact US: help@patsnap.com