EKLPP (Ensemble Kernel Locality Preserving Projections) based fault detection method for continuous process
A technology that maintains projection locally and detects faults. It is applied to computer components, instruments, characters, and pattern recognition. It can solve problems such as different parameters and non-unique faults, and achieve the effect of improving utilization.
- Summary
- Abstract
- Description
- Claims
- Application Information
AI Technical Summary
Problems solved by technology
Method used
Image
Examples
Embodiment Construction
[0015] The present invention will be described in detail below in conjunction with examples.
[0016] The invention uses the Gaussian kernel function to preprocess the continuous process data, and extracts the nonlinear information of the original data. Based on the preprocessing, the local structure of the original data is preserved using locality-preserving projections. By selecting Gaussian kernel functions with different kernel parameters to solve the problem that parameter selection affects fault detection results, multiple sub-models are established, and Bayesian decision-making and ensemble learning methods are used to combine each detection result for continuous process fault detection. This technology solves the problem that the traditional KLPP method selects the same kernel parameters when dealing with different faults.
[0017] Fault detection technology based on integrated kernel local-holding projection: In order to detect process faults, it is necessary to use ...
PUM
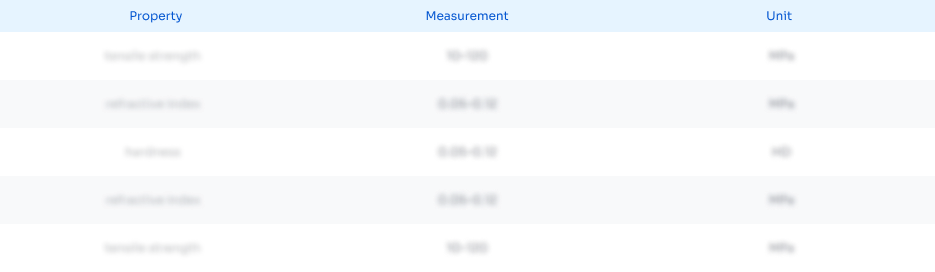
Abstract
Description
Claims
Application Information
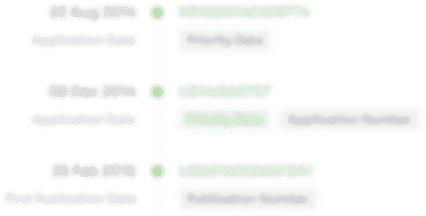
- R&D Engineer
- R&D Manager
- IP Professional
- Industry Leading Data Capabilities
- Powerful AI technology
- Patent DNA Extraction
Browse by: Latest US Patents, China's latest patents, Technical Efficacy Thesaurus, Application Domain, Technology Topic, Popular Technical Reports.
© 2024 PatSnap. All rights reserved.Legal|Privacy policy|Modern Slavery Act Transparency Statement|Sitemap|About US| Contact US: help@patsnap.com