Object identification method based on salient region bag-of-word model
A bag of words model, object recognition technology, applied in character and pattern recognition, instruments, computer parts, etc., can solve the problems of insufficient image feature expression and insufficient quantity.
- Summary
- Abstract
- Description
- Claims
- Application Information
AI Technical Summary
Problems solved by technology
Method used
Image
Examples
Embodiment 1
[0074] The object recognition method based on the salient region bag-of-words model includes three parts: salient region location, feature extraction and description, and image region feature similarity comparison.
[0075] Execute step 100 to perform corner detection.
[0076] ShiTomasi corner points are calculated by calculating the rate of change of the gradient direction, which is the point where the brightness of the image changes drastically or the curvature is very large. The main idea is to use the automatic correlation matrix to determine the change form of the signal in the image. Assuming that the signal at x in the image is I(x), using the image signal and the Gaussian function G(x, σ D ) to perform convolution operation to obtain the first derivative.
[0077] L(x,σ D )=I(x)*G u (x,σ D ) (1)
[0078] L v (x,σ D )=I(x)*G v (x,σ D ) (2)
[0079] L u (x,σ D ) L v (x,σ D ) = I u (x,σ D ) (3)
[0080] where σ D is the differential scale, using the for...
Embodiment 2
[0105] like figure 2 As shown, the similarity comparison of image region features includes the training and testing process of the images in the image library.
[0106] (1) Training process
[0107] Execute step 200, read in the training object picture, and determine the salient region of the image.
[0108] Execute step 210, extract the SIFT feature of the training sample in the salient area, if there are i pieces of training pictures, the number of SIFT feature points of each image is n 1, no 2 ,...,n i , the total number of extracted SIFT features is (n 1 +n 2 +…+n i ).
[0109] Execute step 211, use a size of (n 1 +n 2 +…+n i )×128. The original training matrix is used to store the SIFT features of all samples, and the k-means clustering algorithm is used to create the visual dictionary required by the BOW model. k is the size of the visual dictionary, that is, the dimension of the BOW histogram. Execute step 230, map on the visual dictionary, count the BOW ...
Embodiment 3
[0135] like image 3 As shown, in order to find a stable key point in the multi-scale space, first construct each layer of the pyramid to build a Gaussian difference scale space (DoG):
[0136] D(x,y,σ)=(G(x,y,kσ)-G(x,y,σ))*I(x,y)=L(x,y,kσ)-L(x,y , σ)
[0137] The DoG difference pyramid is obtained from the Gaussian pyramid.
[0138] like Figure 4 As shown, the local extremum points detected on the DoG space are used as key points. In order to find the extremum points, each pixel needs to correspond to 8 adjacent points of the same scale as it and 9×2 adjacent points of the upper and lower adjacent scales. A total of 26 points are compared to ensure that an extreme point is detected in both the scale space and the two-dimensional image space. The pixel point is larger or smaller than the 26 points, that is, it is determined as a key point.
PUM
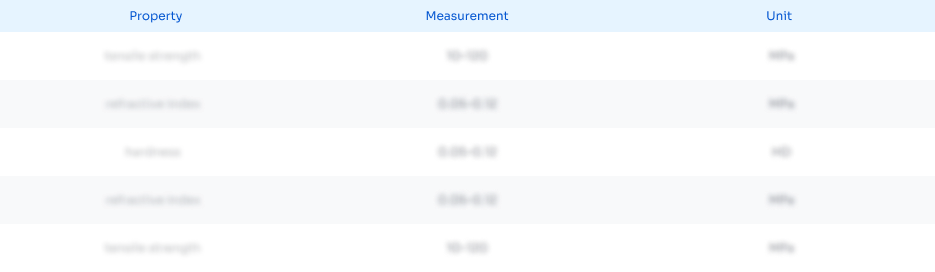
Abstract
Description
Claims
Application Information
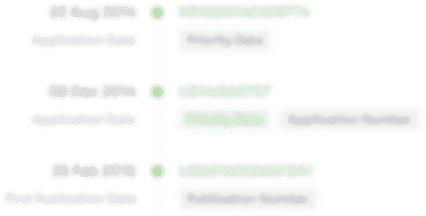
- R&D Engineer
- R&D Manager
- IP Professional
- Industry Leading Data Capabilities
- Powerful AI technology
- Patent DNA Extraction
Browse by: Latest US Patents, China's latest patents, Technical Efficacy Thesaurus, Application Domain, Technology Topic, Popular Technical Reports.
© 2024 PatSnap. All rights reserved.Legal|Privacy policy|Modern Slavery Act Transparency Statement|Sitemap|About US| Contact US: help@patsnap.com