Urban rail transit panoramic monitoring video fault detection method based on depth learning
A technology for urban rail transit and panoramic monitoring, applied in the field of urban rail transit panoramic surveillance video fault detection, to achieve the effect of reducing orders of magnitude, strong generalization ability, and reducing difficulty
- Summary
- Abstract
- Description
- Claims
- Application Information
AI Technical Summary
Problems solved by technology
Method used
Image
Examples
Embodiment 1
[0056] 1. Implementation method of image dataset
[0057] The ultimate purpose of the present invention is to realize the fault recognition of the panoramic monitoring video of urban rail transit, the essence is to realize the recognition of the fault video image, and the image clarity and color cast are two extremely important judgment factors of the video quality detection. The present invention selects pictures with abnormal clarity, pictures with abnormal color cast and normal pictures as sample sets for training and testing, and the images are in RGB color mode. In this process, it is necessary to process the video with abnormal definition, video with abnormal color shift, and normal video, convert the surveillance video into a single frame image (that is, a frame image), and normalize the size of the image to construct an abnormal resolution image. Image Dataset, Color Cast Anomaly Image Dataset, and Normal Image Dataset. According to the distribution ratio, the images ...
Embodiment 2
[0074] The specific steps of the optimization of the fault video image recognition model based on convolutional neural network are as follows:
[0075] Step 1, expand the image dataset
[0076] If the image data set is too small and the extracted features are not sufficient, the abstraction ability of the model for feature learning will be limited, which will affect the test accuracy of the model; the training set with unbalanced positive and negative samples will also lead to inaccurate training results. rate decreased. Therefore, in order to obtain a model with higher accuracy, the present invention expands the 500 images with abnormal definition, 500 images with abnormal color cast, and 500 normal images in the original image data set to 3700 images with abnormal resolution and 4150 images with abnormal color cast. 4,150 normal pictures (the training of the binary classification model needs to ensure that the total number of positive and negative samples exceeds 10,000, of...
PUM
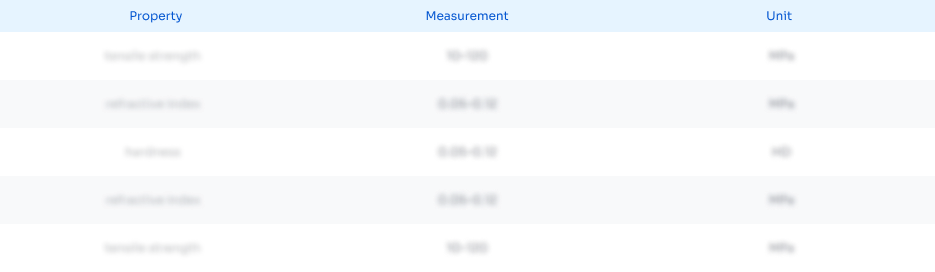
Abstract
Description
Claims
Application Information
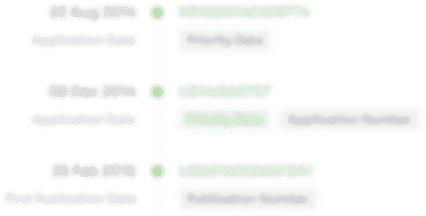
- R&D Engineer
- R&D Manager
- IP Professional
- Industry Leading Data Capabilities
- Powerful AI technology
- Patent DNA Extraction
Browse by: Latest US Patents, China's latest patents, Technical Efficacy Thesaurus, Application Domain, Technology Topic.
© 2024 PatSnap. All rights reserved.Legal|Privacy policy|Modern Slavery Act Transparency Statement|Sitemap