Method for real-time entering passenger flow volume anomaly detection of urban rail transit AFC system
A technology for urban rail transit and anomaly detection, which is applied in the field of intelligent urban rail transit and real-time inbound passenger flow anomaly detection of urban rail transit AFC system. ability, the effect of reducing the false alarm rate
- Summary
- Abstract
- Description
- Claims
- Application Information
AI Technical Summary
Problems solved by technology
Method used
Image
Examples
Embodiment Construction
[0032] The present invention will be further described below in conjunction with the accompanying drawings.
[0033] Such as figure 1 Shown is a real-time inbound passenger flow anomaly detection method for an urban rail transit AFC system. This paper uses the improved small data volume method to calculate the Lyapunov index of the inbound passenger flow time series, and verifies the chaotic characteristics of the sequence; uses the C_C method to calculate the inbound passenger flow time series. Time delay and optimal embedding dimension of passenger flow time series, and phase space reconstruction of the sequence to generate model training, verification and test sample sets; and use the large-scale grid search method to find the parameters of the support vector machine regression model Then use the chaotic support vector machine regression model to predict the inbound passenger flow in each time period, combined with the hypothesis testing method, use the fitting residuals of...
PUM
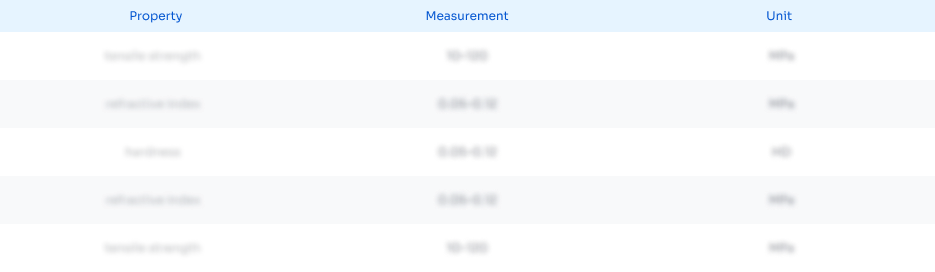
Abstract
Description
Claims
Application Information
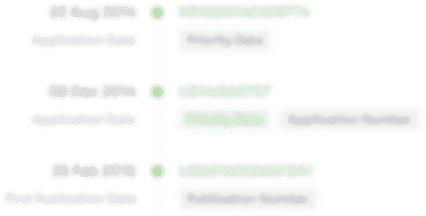
- R&D Engineer
- R&D Manager
- IP Professional
- Industry Leading Data Capabilities
- Powerful AI technology
- Patent DNA Extraction
Browse by: Latest US Patents, China's latest patents, Technical Efficacy Thesaurus, Application Domain, Technology Topic, Popular Technical Reports.
© 2024 PatSnap. All rights reserved.Legal|Privacy policy|Modern Slavery Act Transparency Statement|Sitemap|About US| Contact US: help@patsnap.com