Hyper-spectral image classification method based on recurrent neural network
A recursive neural network and hyperspectral image technology, applied in the field of image processing, can solve the problems of inability to obtain classification effect, general classification effect, affecting classification accuracy, etc., and achieve the effect of improving classification accuracy, improving classification effect, and improving effect.
- Summary
- Abstract
- Description
- Claims
- Application Information
AI Technical Summary
Problems solved by technology
Method used
Image
Examples
Embodiment Construction
[0026] refer to figure 1 , the specific implementation steps of the present invention include:
[0027] Step 1, input hyperspectral image.
[0028] Input a three-dimensional matrix hyperspectral image, the hyperspectral image includes K pixel samples, B hyperspectral bands, and c-type ground objects, where K=K 1 × K 2 , K 1 Indicates the length of the hyperspectral image, K 2 Indicates the width of the hyperspectral image, select 10% of the samples in each type of ground objects as training samples, and the remaining 90% of the samples as test samples.
[0029] Step 2, obtain the spatial texture feature F of the hyperspectral image 1 .
[0030] 2a) Transform the hyperspectral image by using the principal component analysis method, and extract the first k=10 principal component grayscale images;
[0031] 2b) Gabor filters with 4 directions and 3 scales are set, that is, 4 different Gabor kernel function directions and 3 different sinusoidal plane wave wavelengths are set...
PUM
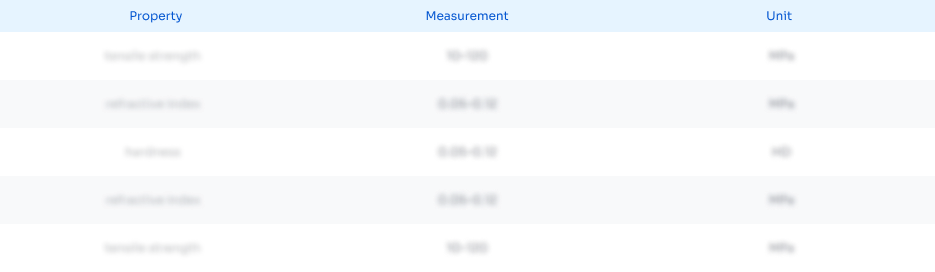
Abstract
Description
Claims
Application Information
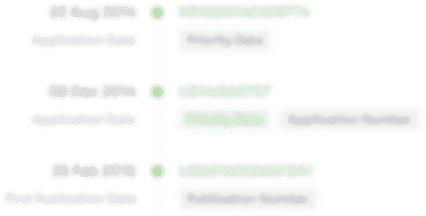
- R&D
- Intellectual Property
- Life Sciences
- Materials
- Tech Scout
- Unparalleled Data Quality
- Higher Quality Content
- 60% Fewer Hallucinations
Browse by: Latest US Patents, China's latest patents, Technical Efficacy Thesaurus, Application Domain, Technology Topic, Popular Technical Reports.
© 2025 PatSnap. All rights reserved.Legal|Privacy policy|Modern Slavery Act Transparency Statement|Sitemap|About US| Contact US: help@patsnap.com