Inversion method of pipeline defect depth based on convolution neural network
A convolutional neural network and defect depth technology, which is applied in the field of pipeline defect depth inversion, can solve the problems of large signal noise and signal distortion, long calculation time, and large result error in the inversion result, so as to improve the inversion accuracy. , reduce the inversion time, the effect of good robustness
- Summary
- Abstract
- Description
- Claims
- Application Information
AI Technical Summary
Problems solved by technology
Method used
Image
Examples
Embodiment Construction
[0040] The specific embodiments of the present invention will be described in detail below in conjunction with the accompanying drawings.
[0041] 1. An inversion method of pipeline defect depth based on convolutional neural network, such as figure 1 shown, including the following steps:
[0042] Step 1: Randomly generate pipeline defect contours: generate n sets of pipeline defect depth matrices D 仿 =(D 1 ,D 2 ,...,D n ), based on the simulation of the magnetic dipole model, the axial magnetic flux leakage signal Y of n sets of pipeline defect profiles is obtained 仿 =(Y 1 , Y 2 ,...,Y n ).
[0043] Step 1.1: Randomly generate a set of pipeline defect contours: pipeline defect length L, pipeline defect width W and its depth matrix D.
[0044] Step 1.1.1: Randomly generate pipe defect length L and pipe defect width W, where L∈L min ~ L max , W∈W min ~W max , L min is the minimum value of randomly generated pipeline defect length, L max is the maximum value of ran...
PUM
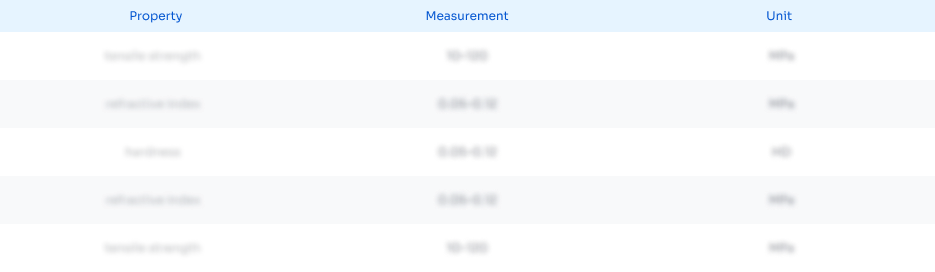
Abstract
Description
Claims
Application Information
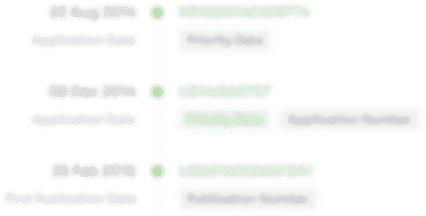
- R&D Engineer
- R&D Manager
- IP Professional
- Industry Leading Data Capabilities
- Powerful AI technology
- Patent DNA Extraction
Browse by: Latest US Patents, China's latest patents, Technical Efficacy Thesaurus, Application Domain, Technology Topic, Popular Technical Reports.
© 2024 PatSnap. All rights reserved.Legal|Privacy policy|Modern Slavery Act Transparency Statement|Sitemap|About US| Contact US: help@patsnap.com