Gesture identification method based on deep residual error network
A gesture recognition and residual technology, applied in biometric recognition, character and pattern recognition, input/output process of data processing, etc., can solve problems such as gradient dispersion
- Summary
- Abstract
- Description
- Claims
- Application Information
AI Technical Summary
Problems solved by technology
Method used
Image
Examples
Embodiment Construction
[0034] In order to make the above-mentioned features and advantages of the present invention more comprehensible, the following specific examples are given and described in detail in conjunction with the accompanying drawings as follows.
[0035] The present invention provides a gesture recognition method based on a deep residual network, such as figure 1 As shown, the method includes a training phase and a recognition phase; the training phase includes the following steps:
[0036] The first step is to obtain the original data information of gestures, in which the present invention acquires 5,000 initial databases for gesture recognition; and after collecting various gesture pictures at the initial stage, mark each gesture picture with N points to obtain 2N-dimensional label data , and save the gesture picture as JPG format, wherein N≥1, and the value of N is mainly determined by the marking personnel; the specific schematic diagram of the gesture picture marking of the prese...
PUM
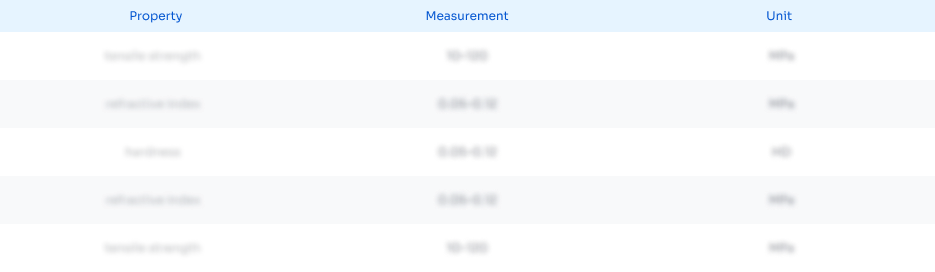
Abstract
Description
Claims
Application Information
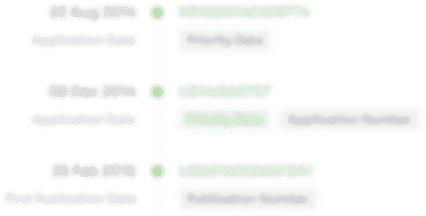
- R&D Engineer
- R&D Manager
- IP Professional
- Industry Leading Data Capabilities
- Powerful AI technology
- Patent DNA Extraction
Browse by: Latest US Patents, China's latest patents, Technical Efficacy Thesaurus, Application Domain, Technology Topic.
© 2024 PatSnap. All rights reserved.Legal|Privacy policy|Modern Slavery Act Transparency Statement|Sitemap