Deep-learning-based Wi-Fi indoor positioning method
An indoor positioning and deep learning technology, applied in neural learning methods, positioning, instruments, etc., can solve the problems of inaccurate positioning, noisy original RSS data, affecting the accuracy and reliability of subsequent positions, and achieve the effect of improving accuracy
- Summary
- Abstract
- Description
- Claims
- Application Information
AI Technical Summary
Problems solved by technology
Method used
Image
Examples
Embodiment 1
[0046] A Wi-Fi indoor positioning method based on deep learning:
[0047] Build the fingerprint library:
[0048] 1) Sample RSS data collection.
[0049] 1-1) Deploy n Wi-Fi wireless access points (APs) in the indoor area where positioning is required.
[0050] 1-2) Divide the indoor area into m domain blocks, where m is a natural number.
[0051] Take the center of each domain block as the sampling point P k , measure the received signal strength RSS of each APs deployed in step 1-1), each sampling point P kThey all correspond to a position coordinate, and the n pieces of RSS data corresponding to a position coordinate form a row of the multidimensional data sample set R after sorting. k=1, 2...m.
[0052] 2) Data preprocessing, completion and normalization.
[0053] 2-1) Perform missing zero padding on the multidimensional data sample set R obtained in step 1).
[0054] 2-1) Normalization processing to obtain the training set H.
[0055] 3) Deep feature learning.
...
Embodiment 2
[0077] In this embodiment, the method described in Embodiment 1 is applied to the positioning of parking spaces in a large complex indoor parking garage.
[0078] It is worth noting that, for large indoor parking garages, finding vehicles has always been a difficult problem to solve. In the prior art (as attached Image 6 As shown), a camera and a license plate recognition system are installed in each parking space to record the parking position of the vehicle. Although this method solves the above-mentioned problems, the cost of installing the camera is very high, and the later maintenance cost and reliability of the camera are not ideal.
[0079] This embodiment discloses a method for finding a vehicle parking position:
[0080] Build the fingerprint library:
[0081] 1) Sample RSS data collection.
[0082] 1-1) If figure 1 As shown, n Wi-Fi wireless access points (APs) are deployed in the indoor garage.
[0083] 1-2) Divide the indoor area into m domain blocks, where...
PUM
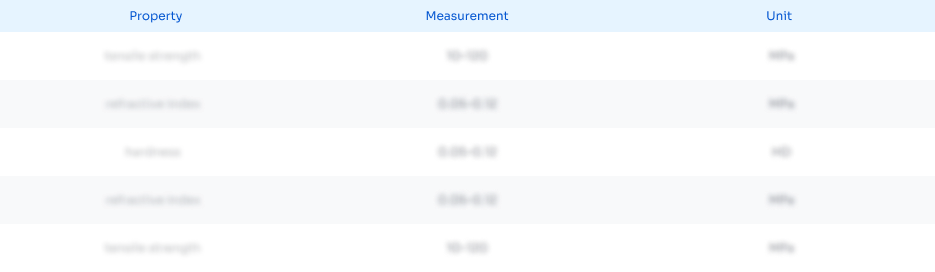
Abstract
Description
Claims
Application Information
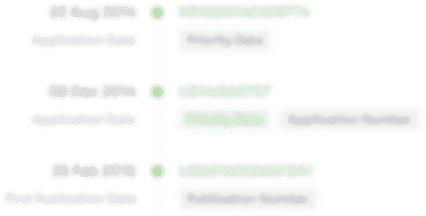
- R&D Engineer
- R&D Manager
- IP Professional
- Industry Leading Data Capabilities
- Powerful AI technology
- Patent DNA Extraction
Browse by: Latest US Patents, China's latest patents, Technical Efficacy Thesaurus, Application Domain, Technology Topic, Popular Technical Reports.
© 2024 PatSnap. All rights reserved.Legal|Privacy policy|Modern Slavery Act Transparency Statement|Sitemap|About US| Contact US: help@patsnap.com