Residual error posterior-based abnormal value online detection and confidence degree assessment method
A technology of confidence and outliers, applied in the field of data monitoring of pollutant emission concentration of coal-fired units, can solve the problems of reducing the reliability of detection methods and lack of prior knowledge of samples
- Summary
- Abstract
- Description
- Claims
- Application Information
AI Technical Summary
Problems solved by technology
Method used
Image
Examples
Embodiment Construction
[0072] The following is based on figure 1 , figure 2 , image 3 with Figure 4 The specific embodiment of the present invention is further described:
[0073] The present invention for a given time series data {x 1 ,x 2 ...,x N}, the general idea of judging whether a new data point x is an abnormal point and evaluating the abnormal confidence of the data point is as follows figure 1 As shown, it can be divided into three stages: model offline training, outlier online identification and model batch update.
[0074] Model offline training stage: build AR prediction model and SOM state model.
[0075] Outlier online identification stage: perform a hypothesis test based on the Bayesian formula on the predicted residual sequence, use the prior probability and conditional probability to calculate the posterior probability that the new data point is a normal point and an abnormal point, and use the two The logarithmic ratio of the posterior probability of is used as an ind...
PUM
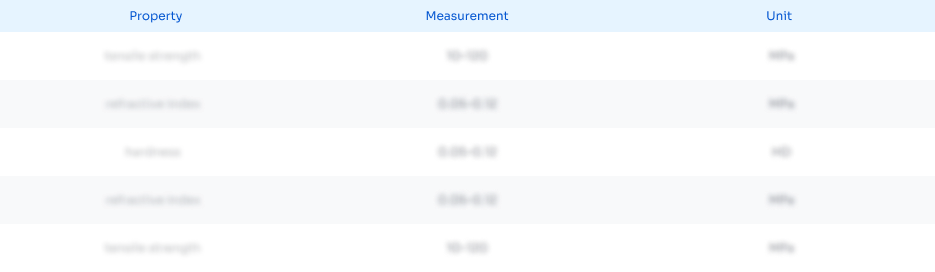
Abstract
Description
Claims
Application Information
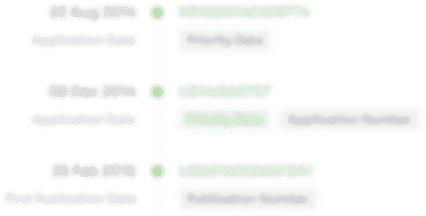
- R&D Engineer
- R&D Manager
- IP Professional
- Industry Leading Data Capabilities
- Powerful AI technology
- Patent DNA Extraction
Browse by: Latest US Patents, China's latest patents, Technical Efficacy Thesaurus, Application Domain, Technology Topic, Popular Technical Reports.
© 2024 PatSnap. All rights reserved.Legal|Privacy policy|Modern Slavery Act Transparency Statement|Sitemap|About US| Contact US: help@patsnap.com