Pulmonary nodule diagnosis method based on double-mode extreme learning machine
A technology of extreme learning machine and pulmonary nodules, which is applied in radiological diagnostic image/data processing, computer components, character and pattern recognition, etc., can solve problems such as unsupervised, unfavorable classification, misdiagnosis, etc. The method is simple and easy to implement Effect
- Summary
- Abstract
- Description
- Claims
- Application Information
AI Technical Summary
Problems solved by technology
Method used
Image
Examples
Embodiment Construction
[0056] The present invention will be described in detail below in conjunction with specific embodiments.
[0057] The deep extreme learning machine self-encoding is composed of N-layer networks, including an input layer and N-1 hidden layers, and the number of neurons in the input layer depends on the size of the input image. In the present invention, the linear interpolation method is used to convert The uniform size of PET-CT images in the dataset is 30×30, so the number of neurons in the input layer is 900. The number of neurons in the hidden layer can be given randomly. In the present invention, 1800 neurons are set in the hidden layer, and the Sigmoid function is used as the activation function of the hidden layer. For images of different modalities, the number of layers of the entire deep network is also different. The number of deep network layers corresponding to CT images is 7 layers, and the number of deep network layers corresponding to PET images is 5 layers.
[0...
PUM
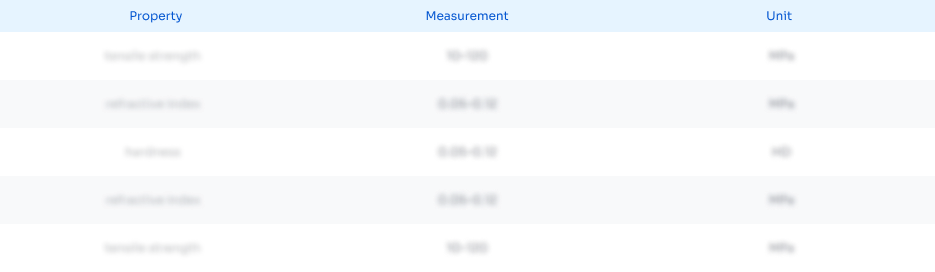
Abstract
Description
Claims
Application Information
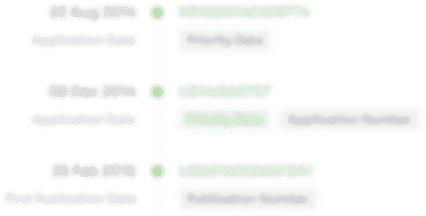
- R&D Engineer
- R&D Manager
- IP Professional
- Industry Leading Data Capabilities
- Powerful AI technology
- Patent DNA Extraction
Browse by: Latest US Patents, China's latest patents, Technical Efficacy Thesaurus, Application Domain, Technology Topic, Popular Technical Reports.
© 2024 PatSnap. All rights reserved.Legal|Privacy policy|Modern Slavery Act Transparency Statement|Sitemap|About US| Contact US: help@patsnap.com