RBF neural network-based coal and gas outburst prediction method
A neural network and gas outburst technology, applied in the field of coal mine safety production, can solve problems such as difficult to determine the optimal parameters of RBF neural network, long training time, falling into local minimum, etc.
- Summary
- Abstract
- Description
- Claims
- Application Information
AI Technical Summary
Problems solved by technology
Method used
Image
Examples
Embodiment Construction
[0054] refer to figure 1 , a coal and gas outburst prediction method based on RBF neural network, proceed as follows:
[0055] Step 1: Obtain a set of training samples of coal and gas outburst, the training samples are made up of feature data X={x 1 ,x 2 ,···,x i ,···,x N} and classification label data Y={y 1 ,y 2 ,···,y i ,···,y N}, where N represents the number of training samples, x i Represents the i-th feature data in the training sample, and has: x i ={x i1 ,x i2 ,···,x iz ,···,x im},x iz Indicates the z-th eigenvalue of the i-th feature data in the training sample, m indicates the dimension of the feature data X; y i Represents the i-th feature data x in the training sample i Corresponding classification labels, and have: y i ={c l |l=1,2,...,C}, C represents the number of classification labels, c l Indicates the l-th classification label, i∈[1,N], z∈[1,m];
[0056] In this embodiment, taking the coal and gas outburst training sample data in Table 1 a...
PUM
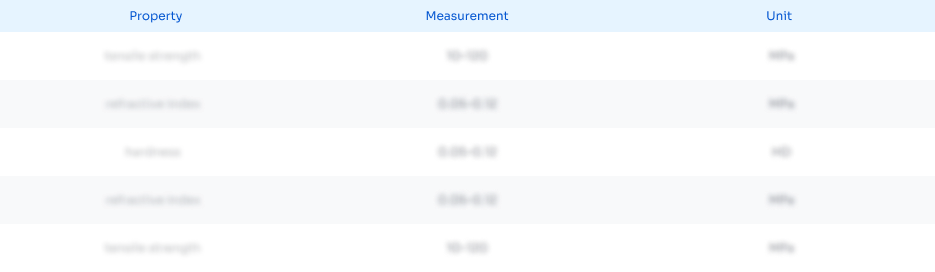
Abstract
Description
Claims
Application Information
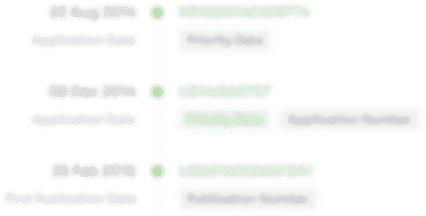
- R&D Engineer
- R&D Manager
- IP Professional
- Industry Leading Data Capabilities
- Powerful AI technology
- Patent DNA Extraction
Browse by: Latest US Patents, China's latest patents, Technical Efficacy Thesaurus, Application Domain, Technology Topic.
© 2024 PatSnap. All rights reserved.Legal|Privacy policy|Modern Slavery Act Transparency Statement|Sitemap