High spectral image object detection method based on tensor principal component analysis dimension reduction
A technology of hyperspectral image and principal component analysis, which is applied in the field of hyperspectral image target detection, can solve the problems of low detection accuracy and inability to mine the overall information of 3D data, and achieve the effect of improving detection accuracy and effective detection
- Summary
- Abstract
- Description
- Claims
- Application Information
AI Technical Summary
Problems solved by technology
Method used
Image
Examples
specific Embodiment approach 1
[0030] Specific implementation mode one: combine figure 1 Describe this embodiment, a hyperspectral image target detection method based on tensor principal component analysis dimensionality reduction in this embodiment, the specific process is:
[0031] Step 1: Select and divide the tensor block of the hyperspectral image to be detected, and obtain the tensor block of the empty X-empty Y-spectrum third-order target template, the tensor block of the third-order background template of the empty X-empty Y-spectrum and the tensor block to be Detected empty X-null Y-spectrum third-order test sample tensor block;
[0032] Step 2: Set the size of the target template tensor block, background template tensor block and test sample tensor block to be tested in each dimension after projection, and use all test sample tensor blocks to obtain the target template tensor block , the projection matrix in three dimensions of the background template tensor block and the test sample tensor bloc...
specific Embodiment approach 2
[0036] Specific embodiment 2: The difference between this embodiment and specific embodiment 1 is that in the first step, the hyperspectral image to be detected is selected and divided into tensor blocks, and the empty X-empty Y-spectrum third-order target template sheet is obtained. Gauge block, empty X-empty Y-spectrum third-order background template tensor block and empty X-empty Y-spectrum third-order test sample tensor block to be detected; the specific process is:
[0037] Given a 3×3 window, convert the hyperspectral image to be detected into a third-order tensor form, slide the sampling window, and determine it as empty X-empty when the value of the truth map corresponding to the center point of the sampling window is 1 Y-spectrum third-order target template tensor block T-tensor(i), i=1,2,...N T ; When the values of all points in the window corresponding to the truth map are 0, it is determined to be empty X-empty Y-spectrum third-order background template tensor bl...
specific Embodiment approach 3
[0039] Specific embodiment three: the difference between this embodiment and specific embodiment one or two is: in the step two, set the target template tensor block, the background template tensor block and each dimension projection of the test sample tensor block to be detected After the size of the dimension, use all the test sample tensor blocks to be detected to obtain the projection matrix in the three dimensions of the target template tensor block, the background template tensor block and the test sample tensor block to be detected; the specific process is:
[0040] Step 21. Set the size P of the projected dimensions of the target template tensor block, the background template tensor block, and the test sample tensor block to be tested. n ,n=1,2,3;
[0041] Step 22: Using the tensor principal component analysis algorithm to use all the test sample tensor blocks to be detected to obtain the projection matrix in the three dimensions of the target template tensor block, th...
PUM
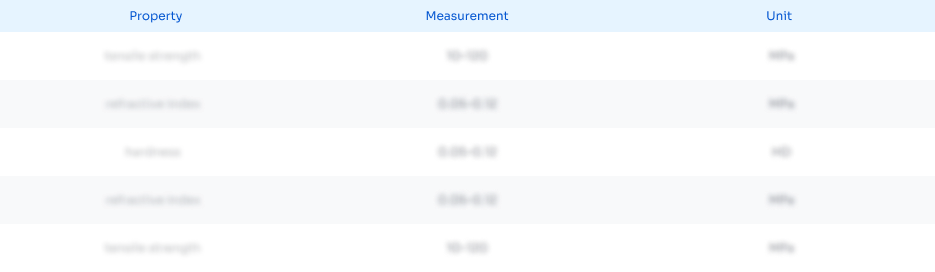
Abstract
Description
Claims
Application Information
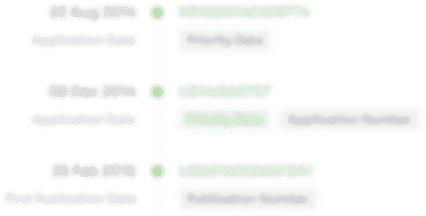
- R&D Engineer
- R&D Manager
- IP Professional
- Industry Leading Data Capabilities
- Powerful AI technology
- Patent DNA Extraction
Browse by: Latest US Patents, China's latest patents, Technical Efficacy Thesaurus, Application Domain, Technology Topic, Popular Technical Reports.
© 2024 PatSnap. All rights reserved.Legal|Privacy policy|Modern Slavery Act Transparency Statement|Sitemap|About US| Contact US: help@patsnap.com