A Mesoscopic Fuel Consumption Prediction Method Based on rbfnn
A prediction method and fuel consumption technology, applied in the direction of neural learning methods, instruments, biological neural network models, etc., can solve the problems of fine-grained monitoring of road microstructure and loss of accuracy of large-scale model calculations, and achieve high precision and generalization The effect of promotion
- Summary
- Abstract
- Description
- Claims
- Application Information
AI Technical Summary
Problems solved by technology
Method used
Image
Examples
Embodiment Construction
[0053] Such as figure 1 As shown, a kind of RBFNN-based mesoscopic fuel consumption prediction method of the present invention is realized through the following steps:
[0054] (1) Determine the influencing factors of road fuel consumption
[0055] The research of the present invention shows that different road section structures have different energy consumption levels under the same speed range.
[0056] The number of lanes is a basic attribute of road structure. The more lanes, the larger the driving space of the driver, so it has a greater impact on the dispersion of driving modes and other characteristics. The lane parameters take values according to the actual number of lanes.
[0057] The lane width also has a great influence on the driver's behavior: if the lane is narrow, the driver tends to widen the distance between the vehicle in front and the lane-changing behavior will be reduced accordingly in order to ensure safety.
[0058] The value method of the lane wi...
PUM
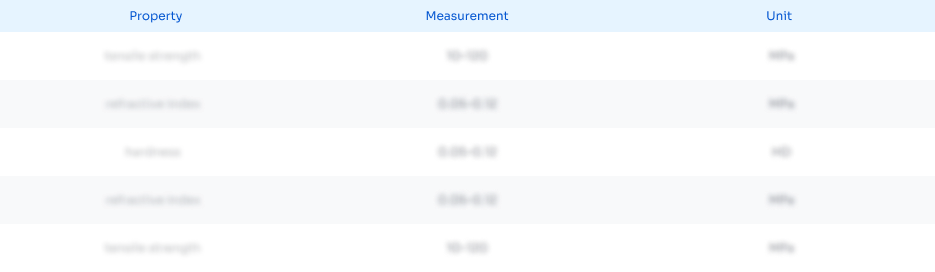
Abstract
Description
Claims
Application Information
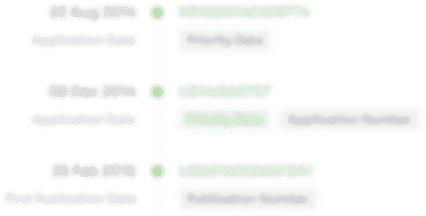
- R&D
- Intellectual Property
- Life Sciences
- Materials
- Tech Scout
- Unparalleled Data Quality
- Higher Quality Content
- 60% Fewer Hallucinations
Browse by: Latest US Patents, China's latest patents, Technical Efficacy Thesaurus, Application Domain, Technology Topic, Popular Technical Reports.
© 2025 PatSnap. All rights reserved.Legal|Privacy policy|Modern Slavery Act Transparency Statement|Sitemap|About US| Contact US: help@patsnap.com